K2VIEW EBOOK
What is Data Mesh?
A Market Primer
Data mesh is a decentralized data management architecture and operating model, which relies on 4 principles: Data products, domain ownership, instant access, and federated governance.
INTRO
Why Data Mesh
The simple premise of data mesh is that business domains should be able to define, access, and control their own data products.
The thinking is that data stakeholders in a specific business domain understand their data needs best. And when when they're forced to work with data teams outside their domain, provisioning the right data to data consumers is time-consuming, often error-prone, and ultimately, ineffective.
Data mesh has emerged to address four key data management challenges:
- A single source of truth is a must, but it’s incredibly challenging when data is fragmented among hundreds of disparate legacy, cloud, and hybrid systems.
- The volume of data is growing exponentially, with increasing demand for instant data access to fresh data.
- Trusted data must be accessible by authorized consumers in the way they need it, without the need for technical expertise or involvement of centralized data teams.
- Effective data management requires collaboration of data engineers, data scientists, business analysts, and operational data consumers.
CHAPTER 01
What is Data Mesh?
Data mesh is an emerging data architecture and operating model for delivering enterprise data, which is founded on 4 key principles:
Data as a product
Data products bundle clean, fresh, and complete data together with everything needed to deliver it safely to authorized data consumers
Data ownership by domains
Business domains create, publish, and manage their own data products, reducing the reliance on centralized data teams
Self-serve data platform
To exercise data autonomy, business domains must be enabled by abstraction and automation that hide underlying data and system complexities
Distributed data governance
Each domain governs its own data products, but remains reliant on centralized data tooling, security policies, and data compliance measures
In a data mesh, every business domain retains control over all aspects of its data products for both analytical and operational workloads – in terms of data quality, freshness, retention, privacy compliance, etc. – and is responsible for sharing them with other domains in the organization.
CHAPTER 02
What are Data Products?
Data products are created to be consumed with a specific purpose in mind. A data product may assume a variety of forms, based on the specific business domain or use case to be addressed.
A data product will often correspond to a dataset of one or more business entities – such as customer, asset, supplier, order, credit card, campaign, etc. – that data consumers would like to access for analytical and operational workloads. The data will typically originate in dozens of siloed source systems, often of different technologies, structures, formats, and terminologies.
A data product, therefore, encapsulates everything that a data consumer requires in order to derive value from the business entity's data. This includes the data product's:
-
Metadata, both static and active (usage and performance)
-
Algorithms, for processing the ingested, raw data (data integration, transformation, cleansing, augmentation, anonymization)
-
Data, ready to be delivered or consumed by authorized users
-
Access methods, such as SQL, JDBC, web services, streaming, CDC,...
-
Synchronization rules, defining how and when the data is synced with the source systems
-
Data governance policies, for data quality and privacy
-
Audit log, of data changes
-
Access controls, including credential checking and authentication
The data product lifecycle
A data product is created by applying cross-functional, product lifecycle methodology to data.
The data product lifecycle adheres to the agile principles of being short and iterative, delivering quick, incremental value to data consumers.
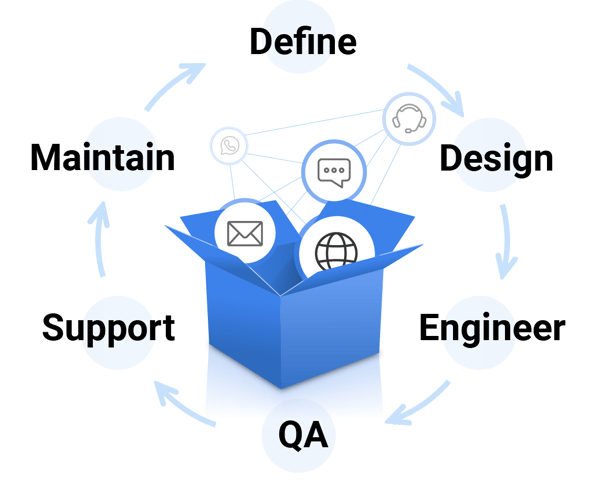
It includes the following phases:
Definition and design
Data product requirements are defined in the context of business objectives, data privacy and governance constraints, and existing data asset inventories. Data product design depends on how the data will be structured, and how it will be componentized as a product, for consumption via services.
Engineering
Data products are engineered by identifying, integrating, and collating the data from its sources, and then employing data masking (aka data anonymization) as needed.
Web service APIs are created to provide consuming applications with the authority to access the data product, and pipelines are secured for delivering the data to its constituents.
Quality assurance
The data is tested and validated to ensure that it’s complete, compliant, and fresh – and that it can be securely consumed by applications at massive scale.
Support and maintenance
Data usage, pipeline performance, and reliability are continually monitored, by local authorities and data engineers, to so issues can be addressed as they arise.
Management
Just as a software product manager is responsible for defining user needs, prioritizing them, and then working with development and QA teams to ensure delivery, the data product approach calls for a similar role. The data product manager is responsible for delivering business value and ROI, where measurable objectives – such as response times for operational insights, or the pace of application development – have definitive goals, or timelines, based on SLAs reached between business and IT.
CHAPTER 03
Attributes of Data Mesh
Decentralization
With the meteoric rise of cloud-based applications, application architectures are transitioning away from centralized IT, towards distributed, data services (or a service mesh).
Data architecture is following the same trend, with data being distributed across a wide range of physical sites, spanning many locations (or a data mesh). Although a monolithic, centralized data architecture is often simpler to create and maintain, in an IT world propelling to the cloud, there are many good reasons and benefits to having a modular, decentralized data management system.
Data mesh represents a decentralized way of distributing data across virtual and physical networks. Where legacy data integration tools require a highly centralized infrastructure, a data mesh operates across on-premise, single-cloud, multi-cloud and edge environments.
Distributed security
When data is highly distributed and decentralized, security plays a critical role. Distributed systems must delegate authentication and authorization activities out to a host of different users, with different levels of access. Key data mesh security capabilities include:
-
Data encryption, at rest and in motion
-
Data masking tools, for effective PII obfuscation
-
Data privacy management, in all its forms
-
GDPR and CCPA compliance, and all other legislation
-
Identity management, including LDAP/IAM-type services
Data product mindset
Innovative data product practices combine the concepts of "design thinking", for breaking down the organizational silos that often impede cross-functional innovation, and the "jobs to be done" theory, which defines the product’s ultimate purpose in fulfilling specific data consumer goals.
CHAPTER 04
Data Mesh Objectives
The objectives of data mesh are to:
-
Exchange data products between data producers and data consumers
-
Simplify the way data is processed, organized, and governed
-
Democratize data with a self-service approach that minimizes dependence on IT
The table below compares the features of traditional data management platforms to data mesh architectures.
Traditional data management platforms | Data mesh architectures |
---|---|
Serve a centralized data team that supports multiple domains | Serve autonomous domain teams |
Manage code, data, and policies, as a single unit | Manage code and pipelines independently |
Require separate stacks for operational and analytical workloads | Provide a single platform for operational and analytic workloads |
Cater to IT, with little regard for Business | Cater to IT and Business, alike |
Centralize the platform for optimized control | Decentralize the platform for optimized scale |
Force domain awareness | Remain domain-agnostic |
The left-hand-side of the table describes most monolithic data platforms. They serve a centralized IT team, and are optimized for control. Operational stacks used to run enterprise software, are completely separated from the clusters managing the analytical data.
The data mesh dictates greater autonomy in the management of data flows, data pipelines, and policies. In the end of the day, data mesh is an architecture based on decentralized thinking that can be applied to any domain.
CHAPTER 05
Data Mesh Challenges
The main challenges of a data mesh stem from the complexities inherent to managing multiple data products (and their dependencies) across multiple autonomous domains.
The key data mesh considerations
Multi-domain data duplication
Redundancy, which may occur when the data of one domain is repurposed to serve the business needs another domain, could potentially impact resource utilization and data management costs.
Federated data governance and quality assurance
Different domains may require different data governance tools, which must be taken into account when data products and pipelines are shared commodities. The resulting deltas must be identified and federated.
Change management
Decentralizing data management to adopt a data mesh approach requires significant change management in highly centralized data management practices.
Cost and risk
Existing data and analytics tools should be adapted and augmented to support a data mesh architecture. Establishing a data management infrastructure to support a data mesh - including data integration, virtualization, preparation, masking, governance, orchestration, cataloging, and delivery - can be a very large, costly, and risky undertaking.
Cross-domain analytics
An enterprise-wide data model must be defined to consolidate the various data products and make them available to authorized users in one central location.
CHAPTER 06
Data Mesh Benefits
The benefits of a data mesh are significant, and include the following:
Agility and scalability
Data mesh improves business domain agility, scalability, and speed to value from data. It decentralizes data operations, and provisions data infrastructure as a service. As a result, it reduces IT backlog, and enables business teams to operate independently and focus on the data products relevant to their needs.
Cross-functional domain teams
As opposed to traditional data architecture approaches, in which highly-skilled technical teams are often involved in creating and maintaining a data pipeline, data mesh puts the control of the data in the hands of the domain experts. With increased IT-business cooperation, domain knowledge is enhanced, and business agility is extended.
Faster data delivery
Data mesh makes data accessible to authorized data consumers in a self-service manner, hiding the underlying data complexities from users.
Strong central governance and compliance
With the ever-growing number of data sources and formats, data lakes and DWHs often fail at massive-scale data integration and ingestion. Domain-based data operations, coupled with strict data governance guidelines, promote easier access to fresh, high-quality data. With data mesh, bulk data dumps into data lakes are things of the past.
CHAPTER 07
Data Mesh Capabilities
Data mesh functional capabilities
Data catalog
Discovers, classifies, and creates and inventory of data assets, and visually displays information supply chains
Data engineering
Rapid creation of scalable and reliable data pipelines that support analytical and operational workloads. Common data preparation flows are productized for reuse by the domains.
Data governance
Distributes certain quality assurance, privacy compliance, and data availability policies and enforcement to the business domains, whilst maintaining centralized governance over company-wide data policies.
Data preparation and orchestration
Enables quick orchestration of source-to-target data flows, including data cleansing, transformation, masking, validation, and enrichment
Data integration and delivery
Accesses data from any source and pipelines it to any target, in any method: ETL (bulk), messaging, CDC, virtualization, and APIs
Data persistence layer
Selectively stores and/or caches data in the hub, or within the domains to improve data access performance
Data mesh non-functional capabilities
Data scale, volume, and performance
Scales both up and down, dynamically, seamlessly, and at high speed, regardless of data volume
Accessibility
Supports all data source types, access modes, formats, technologies, and integrates master and transactional data, at rest, or in motion
Distribution
Deploys on premise, cloud, or in hybrid environments, with complete transactional integrity
Security
Encrypts and masks data, to comply with privacy regulations, and checks user credentials, to ensure authorized access is maintained
CHAPTER 08
Data Mesh Use Cases
Data mesh supports many different operational and analytical use cases, across multiple domains. Here are a few examples:
Customer 360 view, to support customer care in reducing average handle time, increase first contact resolution, and improve customer satisfaction. A single view of the customer may also be deployed by marketing to predictive churn modeling or next-best-offer decisioning
Hyper segmentation, to enable marketing teams deliver the right campaign to the right customer, at the right time, and via the right channel
Data privacy management to protect customer data by complying with ever-emerging regional data privacy laws, like VCDPA, prior to making it available to data consumers in the business domains
IoT device monitoring, providing product teams with insights into edge device usage patterns, to continually improve product adoption and profitability
Federated data preparation, enabling domains to quickly provision quality, trusted data for their data analytics workloads
CHAPTER 09
Implementing Data Mesh with a Data Product Platform
Based on a decentralized design pattern, a real-time data product platform is the optimal implementation for data mesh architecture.
A data product platform creates and delivers data products of connected data from disparate sources to provide a real-time and holistic view of the business to operational and analytical workloads.
A real-time data product platform creates the semantic definition of the various data products that are important to the business. It also sets up the data ingestion methods, and the needed central governance policies, that protect and secure the data in the data products, in accordance with regulations.
Additional platform nodes are deployed in alignment with the business domains, providing the domains with local control of data services and pipelines to access and govern the data products for their respective data consumers.
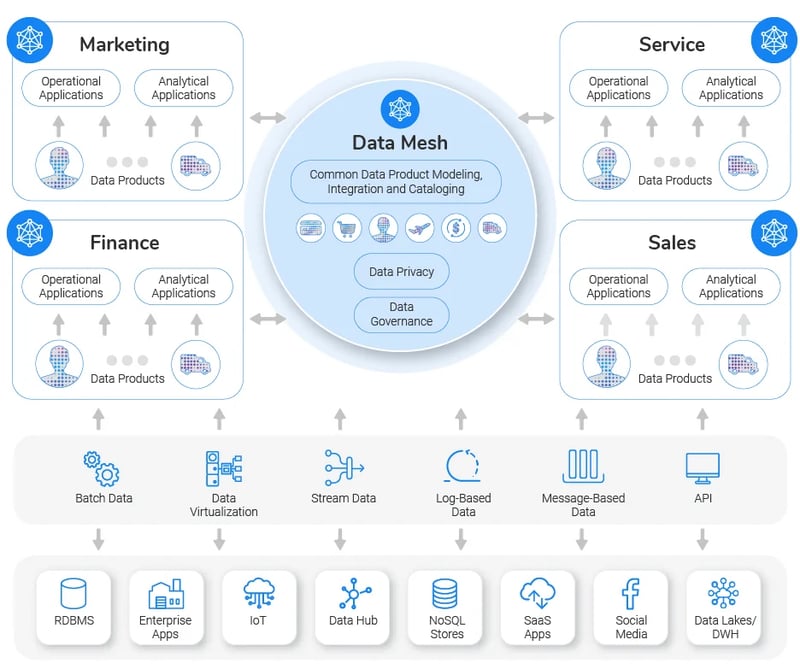
In this sense, a data product platform – that manages, prepares, and delivers data in the form of business entities – becomes the data mesh core.
While data mesh architecture introduces technology and implementation challenges, these are neatly addressed with a data product platform:
Data mesh implementation challenges | How they are addressed by a data product platform |
---|---|
Need for data integration expertise |
Data products as business entities |
Independence vs confederacy |
Cross-functional collaboration |
Real-time and batch data delivery |
Operational and analytical workloads |
CHAPTER 10
K2view Data Product Platform: Data Mesh Inside
The K2view Data Product Platform is ideally suited for implementing a data mesh architecture and operating model because it empowers data teams in business domains to create, govern, and publish their own data products.
The platform provides centralized data modeling, governance, and cataloging, while enabling self-service access to the data by domains – for both analytical and operational workloads.
K2view Data Product Platform uniquely creates a federated alliance across an organization's domains by providing a single, trusted, and holistic view of all business entities that are important to the organization.
These are just some of the reasons that K2view is trusted by some of the world’s largest enterprises as their data product platform of choice.