A practical guide to data masking
What is data masking?
Last updated on March 17, 2025
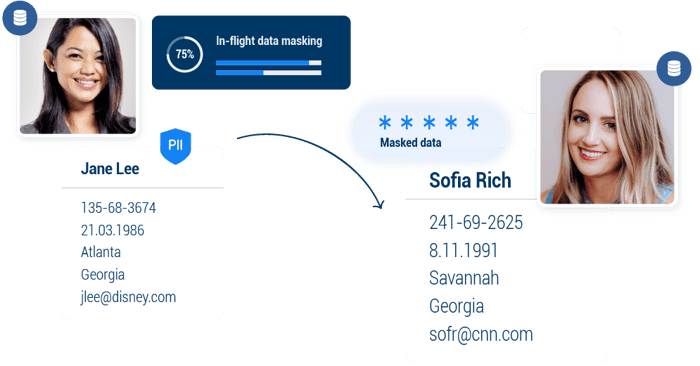
Data masking is the process of permanently concealing PII and other sensitive data, while retaining its referential integrity and semantic consistency.
01
What is data masking?
Data masking is a method for protecting personal or sensitive data that creates a version of the data that can’t be identified or reverse-engineered while retaining referential integrity and usability.
The most common types of data that need masking are:- Personally Identifiable Information (PII) such as names, passport, social security, and telephone numbers
- Protected Health Information (PHI) about an individual’s health status, care, or payment data
- Protected financial data, as mandated by the Payment Card Industry Data Security Standard (PCI-DSS) and the US Federal Trade Commission (FTC) acts and safeguards
- Test data, associated with the Software Development Life Cycle (SDLC)
Masked data is generally used in non-production environments – such as software development and testing, analytics, machine learning, and B2B data sharing – that don’t require the original production data.
Simply defined, data masking combines the processes and tools for making sensitive data unrecognizable to – yet functional for – authorized users.
The data masking process
The data masking process is an iterative lifecycle that can be broken down into 4 steps corresponding to 4 data masking phases – discovering the data, defining the masking rules, deploying the masking functionality, and auditing the entire process on an ongoing basis.
With the right data masking process in place, your data teams can:
- Discover and classify sensitive data
The data masking process begins by analyzing the metadata and data in your enterprise systems and classifying PII and sensitive data that require masking. Sensitive data discovery leverages rules-based and LLM-based techniques to identify the data to be masked. - Define data masking policies
Define the data masking policies for your use cases and set up the functionality to enforce them. Where dynamic data masking is required, define the Role-Based Access Controls (RBAC) that should be applied. Ensure that referential integrity and semantic consistency of the masked data are maintained. - Deploy
A best practice is to deploy data masking tools "close to the source", on premises, so that unmasked data is never transferred to the cloud. Proceed with the necessary security guardrails if data masking is performed in the cloud. - Audit and report for compliance
Generating exportable audit reports – for instance, including schema, table, and column name, as well as type, field, and probability of a match – is an ongoing part of the process.
02
Data masking vs other methods
To understand how data masking works, let’s compare it to data encryption and data tokenization.
While data masking is irreversible, encryption and tokenization are both reversible in the sense that the original values can be derived from the obscured data. Here’s a brief explanation of the 3 methods:
1. Data masking
Data masking tools substitute realistic, but fake data for the original values, to ensure data privacy.
There are many techniques for masking data, such as data scrambling, data blinding, or data shuffling, which will be explained later. Regardless of the method, data masking is irreversible; it's impossible to recover the original values of masked data.
2. Data masking vs. encryption
While data encryption is very secure, data teams can’t analyze or work with encrypted data. The more complex the encryption algorithm, the safer the data will be from unauthorized access. However, while masked data is non-reversal, encrypted data can be decrypted with the right key.
3. Data masking vs. tokenization
Data tokenization, which substitutes a specific sensitive data element with random characters (token), is a reversible process. The token can be mapped back to the original data, with the mappings stored in a secure “data vault”. Any user or application with the right credentials can detokenize the data and access the original data.
Data tokenization supports operations like processing a credit card payment, in support of the Payment Card Industry Data Security Standard (PCI-DSS), without revealing the credit card number. The real data never leaves the organization and can’t be seen or decrypted by a third-party processor.
Data masking is not reversible, making it more secure, and less costly, than tokenization. It maintains referential context and relational integrity across systems and databases, which is critical in data analysis and software testing.
Relational integrity retains data validity and consistency, despite undergoing data de-identification. For example, a real credit card number can be replaced by any 16-digit figure. Once masked and validated, the new value will appear consistently across all systems.
There are 2 major differences between data masking and encryption/tokenization:
Masked data is usable in its anonymized form.
Once data is masked, the original value can’t be recovered.
03
Why is data masking important?
Data masking solutions are important to enterprises because they enable them to:
- Achieve compliance with privacy laws, like CPRA, GDPR and HIPAA, by reducing the risk of exposing personal or sensitive data, as one aspect of the total compliance picture.
- Protect data in lower environments from cyber-attacks, while preserving its usability and consistency.
- Reduce the risk of data sharing, e.g., in the case of cloud migrations, or when integrating with third-party apps.
Masking tools are now needed more than ever before, to effectively safeguard sensitive data and to address the following challenges:
1. Regulatory compliance
Highly regulated industries, like financial services and healthcare, already operate under strict privacy regulations. Besides adhering to regional standards, such as Europe’s GDPR, California’s CPRA, or Brazil’s LGPD, companies in these fields rely on PII data masking to comply with the Payment Card Industry Data Security Standard (PCI DSS), and the Health Insurance Portability and Accountability Act (HIPAA).
And companies aren't keeping up! According to our recent survey, 93% of companies admit that they're not fully compliant:
Test data compliance with data privacy regulations
Source: K2view 2025 State of Test Data Management report
The fact that only 7% of companies say they're fully compliant underscores a critical issue: the widespread presence of PII in test environments in spite of all the legislation to secure sensitive data.
Poor compliance also speaks to the challenges of managing enterprise data across diverse sources and systems, including modern databases such as NoSQL and data lakes and warehouses, as well as unstructured data and mixed environments (cloud and on-prem).
2. Insider threats
Many employees and third-party contractors access enterprise systems on a regular basis, for example for software testing or analytics purposes. Production systems are particularly vulnerable, because sensitive information is often used in development, testing, and other pre-production environments. With insider threats rising 47% since 2018, according to the Ponemon Institute report, protecting sensitive data costs companies an average of $200,000 per year.
3. External threats
In 2020, personal data was compromised in 58% of the data breaches, states a Verizon report. The study further indicates that in 72% of the cases, the victims were large enterprises. With the vast volume, variety and velocity of enterprise data, it is no wonder that breaches proliferate. Taking measures to protect sensitive data in non-production environments will significantly reduce the risk, one of many data masking examples.
4. Data governance
Your data masking tool should be secured with Role-Based Access Control (RBAC). While static data masking obscures a single dataset, dynamic data masking provides more granular controls. With dynamic data masking, permissions can be granted or denied at many different levels. Only those with the appropriate access rights can access the real data. Others will see only the parts that they are allowed to see. You should also be able to apply different masking policies to different users.
04
Data masking use cases
Organizations use data masking to comply with data privacy regulations, like GDPR, CPRA, and HIPAA, mainly to safeguard sensitive data, such as Personally Identifiable Information (PII), Protected Health Information (PHI), and financial data.
Common data masking use cases include:
1. Software development and testing
Software developers and testers often require real data for testing purposes, but access to production datasets is risky. Data masking methods and Test data management tools allow them to work with lifelike test data, without revealing any sensitive information.
2. Analytics and research
With data masking software at their disposal, data analysts and scientists can work with large datasets knowing that confidential information is protected. At the same time, researchers can provide insights by analyzing trends without ever compromising individual privacy.
3. Internal training
By masking data, you can provide real-world examples to your employees without exposing any business or customer data. Your staff can learn and practice skills without having to access any data they’re not authorized to see.
4. External collaboration
Sometimes you need to share data with external consultants, partners, or vendors. With effective PII masking, you can collaborate with third parties without the risk of exposing sensitive data.
5. Retrieval-Augmented Generation (RAG)
Retrieval-Augmented Generation (RAG) is a generative AI framework that's emerging as a key use case for data masking. With RAG, an organization can augment an LLM with its internal data, for more accurate responses. This data must be masked prior to injecting it (as a prompt) into the LLM, to avoid risk of sensitive data leakage.
05
Types of data masking
Over time, many types of data masking have evolved to provide more sophistication, flexibility and security, including:
1. Static data masking
Static data masking, or in-place masking, is a method often used in non-production environments, such as those used for analytics, software testing and development, and end-user training. In such cases, sensitive data is permanently protected in the non-production copy of the data.
Data masking tools ensure that the masked data maintains referential integrity, to enable software development and testing.
2. On-the-fly data masking
The static data masking process introduces risk due to the possible exposure of sensitive data in the staging area, before it is masked.
On-the-fly data masking is performed on data as it moves (in transit) from one environment to another, such as from production, to development or test. This means that the data at rest, in the staging environment, is always compliant.
On-the-fly data masking is ideal for organizations engaging in continuous software delivery, data migrations, and ongoing hydration of data lakes.
3. Dynamic data masking
Dynamic data masking is used to protect, obscure, or block access to sensitive data that's stored in production. Dynamic data masking is performed on the fly, in response to a data request by a certain user or application. When the data is located in multiple source systems, masking consistency is difficult, especially when dealing with disparate environments, and a wide variety of technologies.
Dynamic data masking is used for role-based security – such as handling customer queries, or processing sensitive data, like health records – and in read-only scenarios, so that the masked data doesn’t get written back to the production system.
This technique is frequently used in customer service applications to ensure that support personnel can access the data they need to assist customers while masking sensitive information like credit card numbers or personal identifiers – to maintain privacy and compliance with data protection regulations.
4. Statistical masking
Statistical data masking is method applied in static or on-the-fly masking. It ensures that masked data retains the same statistical characteristics and patterns as the real-world data it represents – such as the distribution, mean, and standard deviation.
5. Unstructured data masking
When it comes to protecting data, regulations do not differentiate between structured and unstructured data. Documents and image files, such as insurance claims, bank checks, and medical records, contain sensitive data. Many different formats (e.g., pdf, png, csv, email, and Office docs) are used daily by enterprises in their regular interactions with individuals. With the potential for so much sensitive data to be exposed in unstructured files, the need for unstructured data masking is obvious.
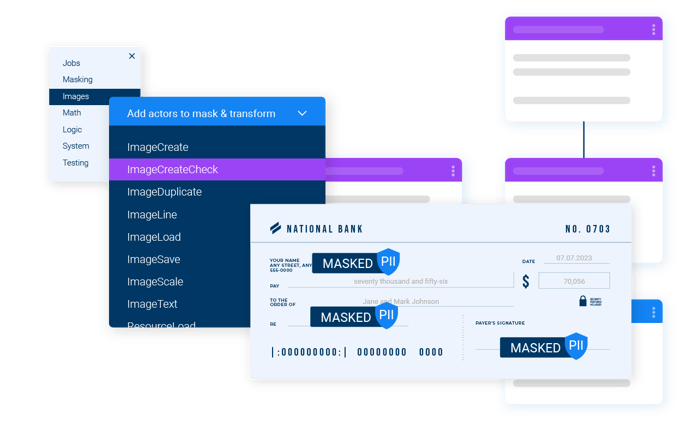
the financial services industry due to strict regulations.
06
Data masking best practices
Here are the most common data masking best practices to assure data security and compliance.
- Leverage the power of AI for PII discovery
Use Large Language Models (LLMs) to complement rules-based methods for accurately locating and classifying PII and sensitive data. -
Classify in a data catalog
Classify your sensitive and PII data, across all your systems and environments, in one centralized data catalog.
-
Determine the right data masking technique
Choose the most appropriate data masking methods for your use case, based on its structure, source, sensitivity, use, and governance policies.
-
Thoroughly test
Verify that your data masking techniques produce the expected results, and that the masked data is realistic, complete, and consistent for your needs.
-
Employ RBAC
Ensure that only authorized personnel can access and modify your data masking algorithms, and that they’re stored and managed securely.
07
Data masking techniques
There are several core data masking techniques associated with data obfuscation, as indicated in the following table:
Technique |
How it works |
Notes |
Data anonymization |
Permanently replaces PII with fake, but realistic, data |
Protects data privacy and supports testing / analytics |
Pseudonymization |
Swaps PII with random values while securely storing the original data when needed |
Applies to unstructured as well as structured data |
Encrypted lookup substitution |
Creates a lookup table with alternative values for PII |
Prevents data breaches by encrypting the table |
Redaction |
Replaces a field containing PII with generic values, completely or partially |
Useful when PII isn’t required or when dynamic data masking is employed |
Shuffling |
Randomly inserts other masked data instead of redacting |
Scrambles the real data in a dataset across multiple records |
Date aging |
Conceals confidential dates by applying random date transformations |
Requires assurance that the new dates are consistent with the rest of the data |
Nulling out |
Protects PII by applying a null value to a data column |
Prevents unauthorized viewing |
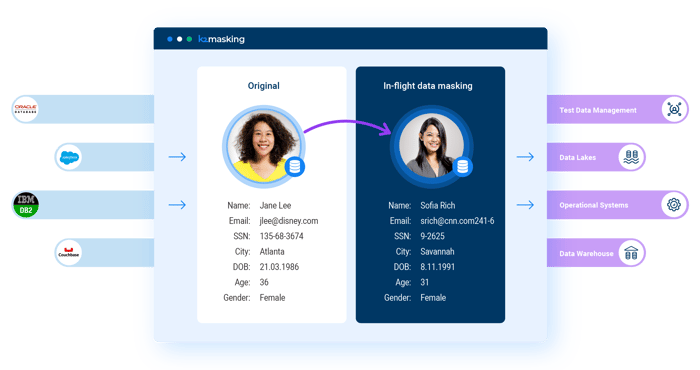
realistic data for software testing and analytics.
08
Data masking challenges
Not only must the altered data retain the basic characteristics of the original data, it must also be transformed enough to eliminate the risk of exposure, while retaining referential integrity.
Enterprise IT landscapes typically have many production systems, that are deployed on premises and in the cloud, across a wide variety of technologies. To mask data effectively, here’s a checklist of must haves:
-
Format preservation
Your data masking tool must understand your data and what it represents. When the real data is replaced with fake data, it should preserve the original format. This capability is essential for data threads that require a specific order, such as dates.
-
Referential integrity
Relational database tables are connected through primary keys. When your masking solution hides or substitutes the values of a table’s primary key, those values must be consistently changed across all databases. For example, if Rick Smith is masked as Sam Jones, that identity must be consistent wherever it resides.
-
PII discovery
PII is scattered across many different databases. The right data masking tool should be able to discover where it’s hiding with advanced capabilities like GenAI-powered PII discovery.
-
Data governance
Data access policies – based on role, location, or permissions – must be established and adhered to.
-
Scalability
Real-time access to structured and unstructured data and mass/batch data extraction must be ensured.
-
Data integration
On-prem or cloud integration with any data source, technology, or vendor is a must, with connections to relational databases, NoSQL sources, legacy systems, message queues, flat files, XML documents, and more.
-
Flexibility
Data masking should be highly customizable. Data teams need to be able to define which data fields are to be masked, and how mask each field.
09
Top data masking tools
Below is a comparison the 6 top data masking tools, listing the strengths and challenges for each:
1. K2view Data Masking
The K2view Data Masking tool is a standalone, best-of-breed solution for enterprises that need to mask data quickly, simply, and at high scale. K2view features structured and unstructured data masking, referential integrity retention, any-source data extraction, auto-discovery of PII, and synthetic data generation capabilities when needed. For all of these reasons, and more, K2view is rated a Visionary by Gartner in its 2024 Magic Quadrant for Data Integration. One user pointed out that K2view was not one of the best known brands, but credibility was quickly established.
2. IBM InfoSphere Optim Data Privacy
IBM® InfoSphere® Optim Data Privacy provides extensive capabilities to effectively mask sensitive data across non-production environments, such as development, testing, QA or training. It provides a variety of transformation techniques that substitute sensitive information with realistic, fully functional masked data. One reviewer said that while it technically does what it is supposed to, the data source integration leaves a lot to be desired.
3. Broadcom Test Data Manager
The Broadcom Test Data Manager helps you address data privacy and compliance Issues as they relate compliance regulations and your corporate mandates. The TDM Discovery and Profiling feature provides the ability to identify personally identifiable information (PII) across multiple data sources. According to reviews, in some areas the product get complicated if the user does not have proper understanding and knowledge.
4. Informatica Cloud Data Masking
Informatica Cloud Data Masking is known for its versatility and compatibility with many different data sources. With broad data masking capabilities, its users are typically organizations with diverse tech stacks. Persistent Data Masking integrates seamlessly with the firm's wider suite, providing a holistic data management approach. However, users complain about high price tags, steep learning curves, and lack of support.
5. OpenText Voltage SecureData Enterprise
The Voltage SecureData Enterprise platform protects any data over its entire lifecycle and helps customers solve complex data privacy challenges. Its format-preserving enterprise data protection techniques include encryption, tokenization, hashing, and masking to address privacy compliance, payments standards and regulations, and data security. Users complain about an outdated UI, the need for better documentation, the lack of data masking capabilities for databases or unstructured data, and the high cost.
6. Delphix DevOps Data Platform
The Delphix DevOps Data Platform masks and provisions data to lower environments, whether it's development, testing, analytics, or AI, providing multi-cloud data automation, compliance, and governance for the application lifecycle. Users are calling for improved security granularity within the Delphix engine, to give specific teams access to their own databases only.
2. IBM InfoSphere Optim Data Privacy
IBM® InfoSphere® Optim Data Privacy provides extensive capabilities to effectively mask sensitive data across non-production environments, such as development, testing, QA or training. It provides a variety of transformation techniques that substitute sensitive information with realistic, fully functional masked data. One reviewer said that while it technically does what it is supposed to, the data source integration leaves a lot to be desired.
3. Broadcom Test Data Manager
The Broadcom Test Data Manager helps you address data privacy and compliance Issues as they relate compliance regulations and your corporate mandates. The TDM Discovery and Profiling feature provides the ability to identify personally identifiable information (PII) across multiple data sources. According to reviews, in some areas the product get complicated if the user does not have proper understanding and knowledge.
4. Informatica Cloud Data Masking
Informatica Cloud Data Masking is known for its versatility and compatibility with many different data sources. With broad data masking capabilities, its users are typically organizations with diverse tech stacks. Persistent Data Masking integrates seamlessly with the firm's wider suite, providing a holistic data management approach. However, users complain about high price tags, steep learning curves, and lack of support.
5. OpenText Voltage SecureData Enterprise
The Voltage SecureData Enterprise platform protects any data over its entire lifecycle and helps customers solve complex data privacy challenges. Its format-preserving enterprise data protection techniques include encryption, tokenization, hashing, and masking to address privacy compliance, payments standards and regulations, and data security. Users complain about an outdated UI, the need for better documentation, the lack of data masking capabilities for databases or unstructured data, and the high cost.
6. Delphix DevOps Data Platform
The Delphix DevOps Data Platform masks and provisions data to lower environments, whether it's development, testing, analytics, or AI, providing multi-cloud data automation, compliance, and governance for the application lifecycle. Users are calling for improved security granularity within the Delphix engine, to give specific teams access to their own databases only.
10
Entity-based data masking
Entity-based data masking resolves the most common data masking challenges, while implementing data masking best practices. It masks all of the sensitive data associated with a specific business entity – e.g., customer, loan, order, or payment – and makes it accessible to authorized data consumers based on role-based access controls.
By taking a business entity approach, data masking ensures both referential integrity and semantic consistency of the masked data. Here's how it works:
- Data is ingested by business entities.
- Sensitive data is masked by entity, maintaining referential integrity and semantic consistency.
- The masked dataset is delivered to downstream systems, by business entities.
For example, if customer data is stored in 4 different source systems (let's say orders, invoices, payments, and service tickets), then entity-based data masking ingests and unifies customer data from the 4 systems to create a "golden record" for each customer.
The PII data associated with the individual customer is masked consistently, and the anonymized customer data is provisioned to the downstream systems or data stores.
Moreover, if, for example, the customer's status was masked to "VIP", which requires a certain payment threshold to have been exceeded, then the customer's payments are increased accordingly to ensure semantic consistency with the VIP status.
This makes entity-based data masking ideal for data analytics, software testing, training, and retrieval augmented generation use cases.
The entity-based approach supports structured and unstructured data masking, static and dynamic data masking, test data masking, and more. Images, PDFs, text, and XML files that may contain PII are protected, while operational and analytical workloads continue to run without interruption.
If you’d like to leverage the latest data masking capabilities and want to avoid the vulnerabilities of conventional methods, a business entity approach is the right way to go.
By managing data with a business entity approach,
referential integrity and consistency are ensured.
Complimentary DOWNLOAD
Free Gartner Report: Market Guide for Data Masking
Learn all about data masking from industry analyst Gartner:
-
Market description, including dynamic and static data masking techniques
-
Critical capabilities, such as PII discovery, rule management, operations, and reporting
-
Data masking vendors, broken down by category
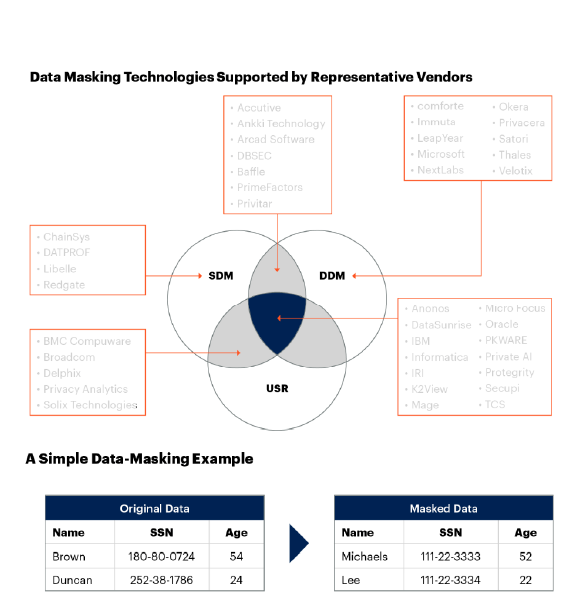