A practical guide to data masking
What is data masking?
Last updated on December 10, 2024
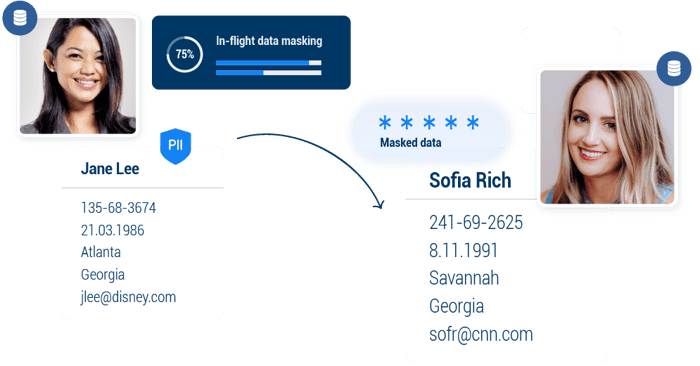
Data masking is the process of permanently concealing PII and other sensitive data, while retaining its referential integrity and semantic consistency.
01
What is data masking?
Data masking is a method for protecting personal or sensitive data that creates a version of the data that can’t be identified or reverse-engineered while retaining referential integrity and usability.
The most common types of data that need masking are:- Personally Identifiable Information (PII) such as names, passport, social security, and telephone numbers
- Protected Health Information (PHI) about an individual’s health status, care, or payment data
- Protected financial data, as mandated by the Payment Card Industry Data Security Standard (PCI-DSS) and the US Federal Trade Commission (FTC) acts and safeguards
- Test data, associated with the Software Development Life Cycle (SDLC)
Masked data is generally used in non-production environments – such as software development and testing, analytics, machine learning, and B2B data sharing – that don’t require the original production data.
Simply defined, data masking combines the processes and tools for making sensitive data unrecognizable to – yet functional for – authorized users.
The data masking process
The data masking process is an iterative lifecycle that can be broken down into 4 steps corresponding to 4 data masking phases – discovering the data, defining the masking rules, deploying the masking functionality, and auditing the entire process on an ongoing basis.
With the right data masking process in place, your data teams can:
- Discover and classify sensitive data
The data masking process begins by analyzing the metadata and data in your enterprise systems and classifying PII and sensitive data that require masking. Sensitive data discovery leverages rules-based and LLM-based techniques to identify the data to be masked. - Define data masking policies
Define the data masking policies for your use cases and set up the functionality to enforce them. Where dynamic data masking is required, define the Role-Based Access Controls (RBAC) that should be applied. Ensure that referential integrity and semantic consistency of the masked data are maintained. - Deploy
A best practice is to deploy data masking tools "close to the source", on premises, so that unmasked data is never transferred to the cloud. Proceed with the necessary security guardrails if data masking is performed in the cloud. - Audit and report for compliance
Generating exportable audit reports – for instance, including schema, table, and column name, as well as type, field, and probability of a match – is an ongoing part of the process.
02
Data masking vs other methods
To understand how data masking works, let’s compare it to data encryption and data tokenization.
While data masking is irreversible, encryption and tokenization are both reversible in the sense that the original values can be derived from the obscured data. Here’s a brief explanation of the 3 methods:
Data masking
Data masking tools substitute realistic, but fake data for the original values, to ensure data privacy.
There are many techniques for masking data, such as data scrambling, data blinding, or data shuffling, which will be explained later. Regardless of the method, data masking is irreversible; it's impossible to recover the original values of masked data.
Data masking vs. encryption
While data encryption is very secure, data teams can’t analyze or work with encrypted data. The more complex the encryption algorithm, the safer the data will be from unauthorized access. However, while masked data is non-reversal, encrypted data can be decrypted with the right key.
Data masking vs. tokenization
Data tokenization, which substitutes a specific sensitive data element with random characters (token), is a reversible process. The token can be mapped back to the original data, with the mappings stored in a secure “data vault”. Any user or application with the right credentials can detokenize the data and access the original data.
Data tokenization supports operations like processing a credit card payment, in support of the Payment Card Industry Data Security Standard (PCI-DSS), without revealing the credit card number. The real data never leaves the organization and can’t be seen or decrypted by a third-party processor.
Data masking is not reversible, making it more secure, and less costly, than tokenization. It maintains referential context and relational integrity across systems and databases, which is critical in data analysis and software testing .
Relational integrity retains data validity and consistency, despite undergoing data de-identification. For example, a real credit card number can be replaced by any 16-digit figure. Once masked and validated, the new value will appear consistently across all systems.
There are 2 major differences between data masking and encryption/tokenization:
Masked data is usable in its anonymized form.
Once data is masked, the original value can’t be recovered.
03
Why is data masking important?
Data masking solutions are important to enterprises because they enable them to:
- Achieve compliance with privacy laws, like CPRA, GDPR and HIPAA, by reducing the risk of exposing personal or sensitive data, as one aspect of the total compliance picture.
- Protect data in lower environments from cyber-attacks, while preserving its usability and consistency.
- Reduce the risk of data sharing, e.g., in the case of cloud migrations, or when integrating with third-party apps.
Masking tools are now needed more than ever before, to effectively safeguard sensitive data and to address the following challenges:
Regulatory compliance
Highly regulated industries, like financial services and healthcare, already operate under strict privacy regulations. Besides adhering to regional standards, such as Europe’s GDPR, California’s CPRA, or Brazil’s LGPD, companies in these fields rely on PII data masking to comply with the Payment Card Industry Data Security Standard (PCI DSS), and the Health Insurance Portability and Accountability Act (HIPAA).
Insider threats
Many employees and third-party contractors access enterprise systems on a regular basis, for example for software testing or analytics purposes. Production systems are particularly vulnerable, because sensitive information is often used in development, testing, and other pre-production environments. With insider threats rising 47% since 2018, according to the Ponemon Institute report, protecting sensitive data costs companies an average of $200,000 per year.
External threats
In 2020, personal data was compromised in 58% of the data breaches, states a Verizon report. The study further indicates that in 72% of the cases, the victims were large enterprises. With the vast volume, variety and velocity of enterprise data, it is no wonder that breaches proliferate. Taking measures to protect sensitive data in non-production environments will significantly reduce the risk, one of many data masking examples.
Data governance
Your data masking tool should be secured with Role-Based Access Control (RBAC). While static data masking obscures a single dataset, dynamic data masking provides more granular controls. With dynamic data masking, permissions can be granted or denied at many different levels. Only those with the appropriate access rights can access the real data. Others will see only the parts that they are allowed to see. You should also be able to apply different masking policies to different users.
04
Data masking use cases
Organizations use data masking to comply with data privacy regulations, like GDPR, CPRA, and HIPAA, mainly to safeguard sensitive data, such as Personally Identifiable Information (PII), Protected Health Information (PHI), and financial data.
Common data masking use cases include:
Software development and testing
Software developers and testers often require real data for testing purposes, but access to production datasets is risky. Data masking methods and Test data management tools allow them to work with lifelike test data, without revealing any sensitive information.
Analytics and research
With data masking software at their disposal, data analysts and scientists can work with large datasets knowing that confidential information is protected. At the same time, researchers can provide insights by analyzing trends without ever compromising individual privacy.
Internal training
By masking data, you can provide real-world examples to your employees without exposing any business or customer data. Your staff can learn and practice skills without having to access any data they’re not authorized to see.
External collaboration
Sometimes you need to share data with external consultants, partners, or vendors. With effective PII masking, you can collaborate with third parties without the risk of exposing sensitive data.
Retrieval-augmented generation
Retrieval-Augmented Generation (RAG) is a generative AI framework that's emerging as a key use case for data masking. With RAG, an organization can augment an LLM with its internal data, for more accurate responses. This data must be masked prior to injecting it (as a prompt) into the LLM, to avoid risk of sensitive data leakage.
05
Types of data masking
Over time, many types of data masking have evolved to provide more sophistication, flexibility and security, including:
Static data masking
Static data masking, or in-place masking, is a method often used in non-production environments, such as those used for analytics, software testing and development, and end-user training. In such cases, sensitive data is permanently protected in the non-production copy of the data.
Data masking tools ensure that the masked data maintains referential integrity, to enable software development and testing.
On-the-fly data masking
The static data masking process introduces risk due to the possible exposure of sensitive data in the staging area, before it is masked.
On-the-fly data masking is performed on data as it moves (in transit) from one environment to another, such as from production, to development or test. This means that the data at rest, in the staging environment, is always compliant.
On-the-fly data masking is ideal for organizations engaging in continuous software delivery, data migrations, and ongoing hydration of data lakes.
Dynamic data masking
Dynamic data masking is used to protect, obscure, or block access to sensitive data that's stored in production. Dynamic data masking is performed on the fly, in response to a data request by a certain user or application. When the data is located in multiple source systems, masking consistency is difficult, especially when dealing with disparate environments, and a wide variety of technologies.
Dynamic data masking is used for role-based security – such as handling customer queries, or processing sensitive data, like health records – and in read-only scenarios, so that the masked data doesn’t get written back to the production system.
This technique is frequently used in customer service applications to ensure that support personnel can access the data they need to assist customers while masking sensitive information like credit card numbers or personal identifiers – to maintain privacy and compliance with data protection regulations.
Statistical masking
Statistical data masking is method applied in static or on-the-fly masking. It ensures that masked data retains the same statistical characteristics and patterns as the real-world data it represents – such as the distribution, mean, and standard deviation.
Unstructured data masking
When it comes to protecting data, regulations do not differentiate between structured and unstructured data. Documents and image files, such as insurance claims, bank checks, and medical records, contain sensitive data. Many different formats (e.g., pdf, png, csv, email, and Office docs) are used daily by enterprises in their regular interactions with individuals. With the potential for so much sensitive data to be exposed in unstructured files, the need for unstructured data masking is obvious.
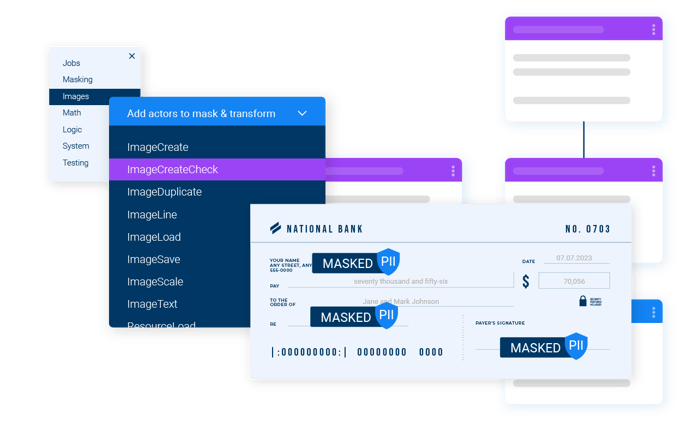
the financial services industry due to strict regulations.
06
Data masking best practices
Here are the most common data masking best practices to assure data security and compliance.
- Leverage the power of AI for PII discovery
Use Large Language Models (LLMs) to complement rules-based methods for accurately locating and classifying PII and sensitive data. -
Classify in a data catalog
Classify the your sensitive and PII data, across your your systems and environments, in a centralized data catalog.
-
Determine the right data masking technique
Choose the most appropriate data masking methods for your use case, based on its structure, source, sensitivity, use, and governance policies.
-
Thoroughly test
Verify that your data masking techniques produce the expected results, and that the masked data is realistic, complete, and consistent for your needs.
-
Employ RBAC
Ensure that only authorized personnel can access and modify your data masking algorithms, and that they’re stored and managed securely.
07
Data masking techniques
There are several core data masking techniques associated with data obfuscation, as indicated in the following table:
Technique |
How it works |
Notes |
Data anonymization |
Permanently replaces PII with fake, but realistic, data |
Protects data privacy and supports testing / analytics |
Pseudonymization |
Swaps PII with random values while securely storing the original data when needed |
Applies to unstructured as well as structured data |
Encrypted lookup substitution |
Creates a lookup table with alternative values for PII |
Prevents data breaches by encrypting the table |
Redaction |
Replaces a field containing PII with generic values, completely or partially |
Useful when PII isn’t required or when dynamic data masking is employed |
Shuffling |
Randomly inserts other masked data instead of redacting |
Scrambles the real data in a dataset across multiple records |
Date aging |
Conceals confidential dates by applying random date transformations |
Requires assurance that the new dates are consistent with the rest of the data |
Nulling out |
Protects PII by applying a null value to a data column |
Prevents unauthorized viewing |
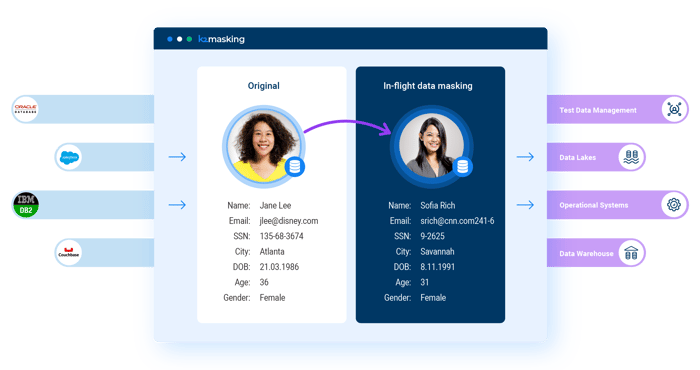
realistic data for software testing and analytics.
08
Data masking challenges
Not only must the altered data retain the basic characteristics of the original data, it must also be transformed enough to eliminate the risk of exposure, while retaining referential integrity.
Enterprise IT landscapes typically have many production systems, that are deployed on premises and in the cloud, across a wide variety of technologies. To mask data effectively, here’s a checklist of must haves:
-
Format preservation
Your data masking tool must understand your data and what it represents. When the real data is replaced with fake data, it should preserve the original format. This capability is essential for data threads that require a specific order, such as dates.
-
Referential integrity
Relational database tables are connected through primary keys. When your masking solution hides or substitutes the values of a table’s primary key, those values must be consistently changed across all databases. For example, if Rick Smith is masked as Sam Jones, that identity must be consistent wherever it resides.
-
PII discovery
PII is scattered across many different databases. The right data masking tool should be able to discover where it’s hiding with advanced capabilities like GenAI-powered PII discovery.
-
Data governance
Data access policies – based on role, location, or permissions – must be established and adhered to.
-
Scalability
Real-time access to structured and unstructured data and mass/batch data extraction must be ensured.
-
Data integration
On-prem or cloud integration with any data source, technology, or vendor is a must, with connections to relational databases, NoSQL sources, legacy systems, message queues, flat files, XML documents, and more.
-
Flexibility
Data masking should be highly customizable. Data teams need to be able to define which data fields are to be masked, and how mask each field.
09
Top data masking tools
Below is a comparison the 6 top data masking tools, listing the strengths and challenges for each:
1. K2view Data Masking
K2view Data Masking tools are built in to its data product platform. The company’s patented Micro-Database™ technology organizes data by individual business entities (e.g., customers, devices, invoices, or orders). Designed for large enterprises that need to mask data quickly, simply, and at high scale, K2view also masks both structured and unstructured data in flight, while retaining referential integrity. According to Gartner Peer Insights K2view features "expedited deployment times, scaling capabilities, and adaptability to alterations," but is mostly used by companies requiring substantial data management solutions.
2. EPI-USE LABS for SAP
EPI-USE LABS are focused on data masking for SAP environments. Its integration with SAP systems protects data within this specific domain. While users claim that their tool is simple and transparent, they also say that the interface could be clearer – and that they need different tools for their non-SAP databases.
3. Oracle Data Masking and Subsetting
Oracle Data Masking and Subsetting tool is a data protection solution for Oracle ecosystems. Its integration with Oracle databases facilitates masking, but reviewers operating in multi-database environments report that the platform is very complex, even for expert users – and that their non-Oracle databases require other tools.
4. IBM InfoSphere Optim Data Privacy
IBM InfoSphere Optim Data Privacy tool offers data masking for companies with complex data environments. Its test data subsetting feature makes it suitable for organizations with diverse data sources. Users complain of an antiquated user interface, functionality gaps, and inadequate data source integration.
5. Camouflage Software
Camouflage Software differentiates itself with with user-friendly interfaces and do-it-yourself implementation. Although it supports many different databases and file formats, users operating in highly specialized or complex environments find its feature set lacking.
6. Informatica Cloud Data Masking
Informatica Cloud Data Masking is known for its versatility and compatibility with many different data sources. With broad data masking capabilities, its users are typically organizations with diverse tech stacks. Persistent Data Masking integrates seamlessly with the firm's wider suite, providing a holistic data management approach. However, users complain about high price tags, steep learning curves, and lack of support.
10
Entity-based data masking
Entity-based data masking resolves the most common data masking challenges, while implementing data masking best practices. It masks all of the sensitive data associated with a specific business entity – e.g., customer, loan, order, or payment – and makes it accessible to authorized data consumers based on role-based access controls.
By taking a business entity approach, data masking ensures both referential integrity and semantic consistency of the masked data. Here's how it works:
- Data is ingested by business entities.
- Sensitive data is masked by entity, maintaining referential integrity and semantic consistency.
- The masked dataset is delivered to downstream systems, by business entities.
For example, if customer data is stored in 4 different source systems (let's say orders, invoices, payments, and service tickets), then entity-based data masking ingests and unifies customer data from the 4 systems to create a "golden record" for each customer. The PII data associated with the individual customer is masked consistently, and the anonymized customer data is provisioned to the downstream systems or data stores. Moreover, if, for example, the customer's status was masked to "VIP", which requires a certain payment threshold to have been exceeded, then the customer's payments are increased accordingly to ensure semantic consistency with the VIP status.
This makes entity-based data masking ideal for data analytics, software testing, training, and retrieval augmented generation use cases.
The entity-based approach supports structured and unstructured data masking, static and dynamic data masking, test data masking, and more. Images, PDFs, text, and XML files that may contain PII are protected, while operational and analytical workloads continue to run without interruption.
If you’d like to leverage the latest data masking capabilities and want to avoid the vulnerabilities of conventional methods, a business entity approach is the right way to go.
By managing data with a business entity approach, referential integrity and consistency are ensured.
Complimentary DOWNLOAD
Free Gartner Report: Market Guide for Data Masking
Learn all about data masking from industry analyst Gartner:
-
Market description, including dynamic and static data masking techniques
-
Critical capabilities, such as PII discovery, rule management, operations, and reporting
-
Data masking vendors, broken down by category
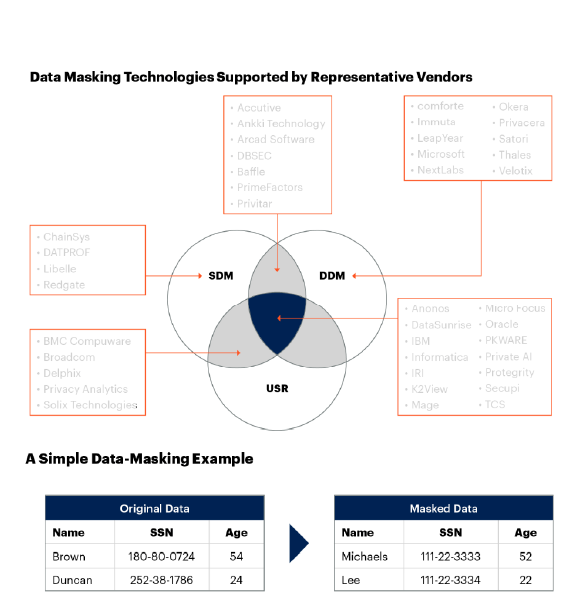