Data fabric is a centralized data architecture that serves authorized consumers with integrated, governed, fresh data – for analytical and operational workloads.
Over the past few years, the term “data fabric” has become synonymous with enterprise data integration and management. Analyst firm Gartner lists “data fabric” as a “Top strategic technology trend” and predicts that by 2024, 25% of data management vendors will provide a complete framework for data fabric – up from 5% today.
Data Fabric Definition
Data fabric democratizes data access across the enterprise, at scale. It is a single, unified architecture – with an integrated set of technologies and services, designed to deliver integrated and enriched data – at the right time, in the right method, and to the right data consumer – in support of both operational and analytical workloads.
Data fabric combines key data management technologies – such as data catalog, data governance, data integration, data pipelining, and data orchestration.
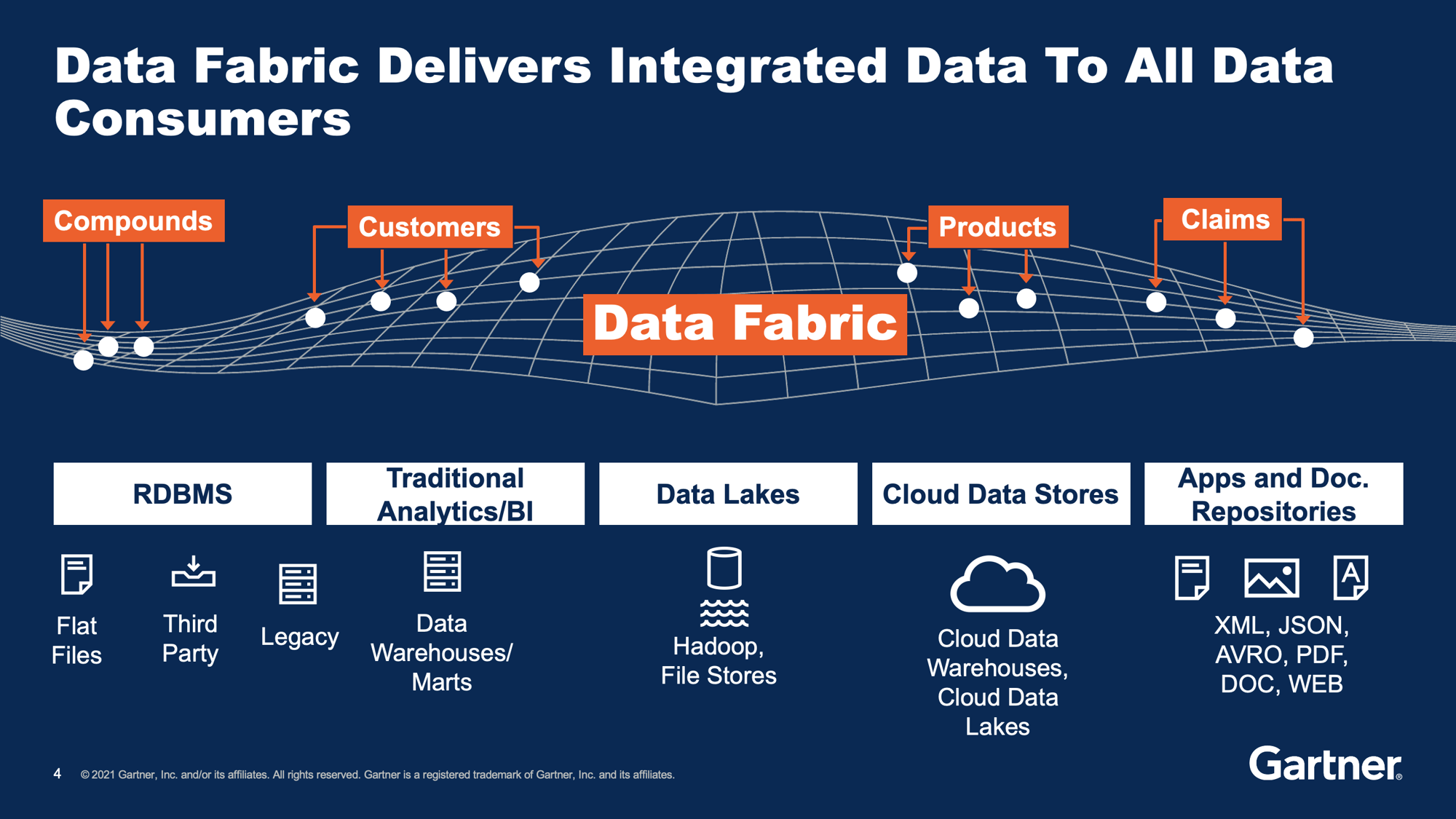
Why Data Fabric
Data fabric serves a broad range of business, technical, and organizational alignment drivers.
Business drivers
-
Quicker time to insights and decision-making, by pipelining data into data lakes and warehouses reliably and quickly.
-
Real-time, 360-degree view of any business entity – such as a customer, claim, order, device, or retail outlet – to achieve micro-segmentation, reduce customer churn, alert on operational risks, or deliver personalized customer service.
-
Reduced total cost of ownership – to operate, scale, maintain, and change legacy systems –by modernizing them incrementally and quickly.
Data management drivers
-
Data preparation automation saves data scientists, data engineers, and other IT resources, from undertaking tedious repetitive data transformation, cleansing, and enrichment tasks.
-
Access to enterprise data in any data delivery method – including bulk data movement (ETL), data virtualization, data streaming, change data capture, and APIs.
-
A data fabric platform integrates and augments a company’s data management tools currently in use, and enables the retirement of others, for increased cost effectiveness.
Organizational drivers
-
A common language shared between data engineers and data consumers improves collaboration between data and business teams.
-
Self-service data access capabilities let data consumers get the data they need, whenever they need it, for increased business agility and speed.
Data Fabric Architecture
Gartner: An ideal, complete data fabric design with its many components.
A well designed data fabric architecture is modular and supports massive scale, distributed multi-cloud, on-premise, and hybrid deployment.
As the diagram above illustrates, as data is provisioned from sources to consumers, it is cataloged, prepared, enriched to provide insights and recommendations, orchestrated, and delivered.
The data fabric is able to integrate and unify data from all data sources, ranging from siloed legacy systems to modern cloud applications and analytical datastores (data warehouses and lakes).
Data consumers of the data fabric include analytical data users such as data scientists and data analysts, as well as operational workloads such as MDM, Customer 360, test data management, and more.
Data Fabric and Data Mesh Architectures
Data mesh architecture addresses the four key issues in data management:
-
Data scattered among dozens, and at times hundreds, of legacy and cloud systems, making it difficult to achieve a single source of truth
-
Speed and volume of data, that data-centric enterprises have to deal with
-
Data hard to understand unless you are a line of business SME
-
Lack of communication between business analysts, operational data consumers, data engineers, and data scientists.
Data mesh is a distributed data management architecture and operational model that provides data product ownership to business domains.
Data fabric complements data mesh because it builds an integrated layer of connected data across a broad range of data sources. It provides an instant, holistic view of the business for both analytical and operational workloads.
Data fabric establishes the semantic definition of the different data products, the data ingestion modes, and the necessary governance policies that secure and protect the data. It functions as the hub in a data mesh architecture.
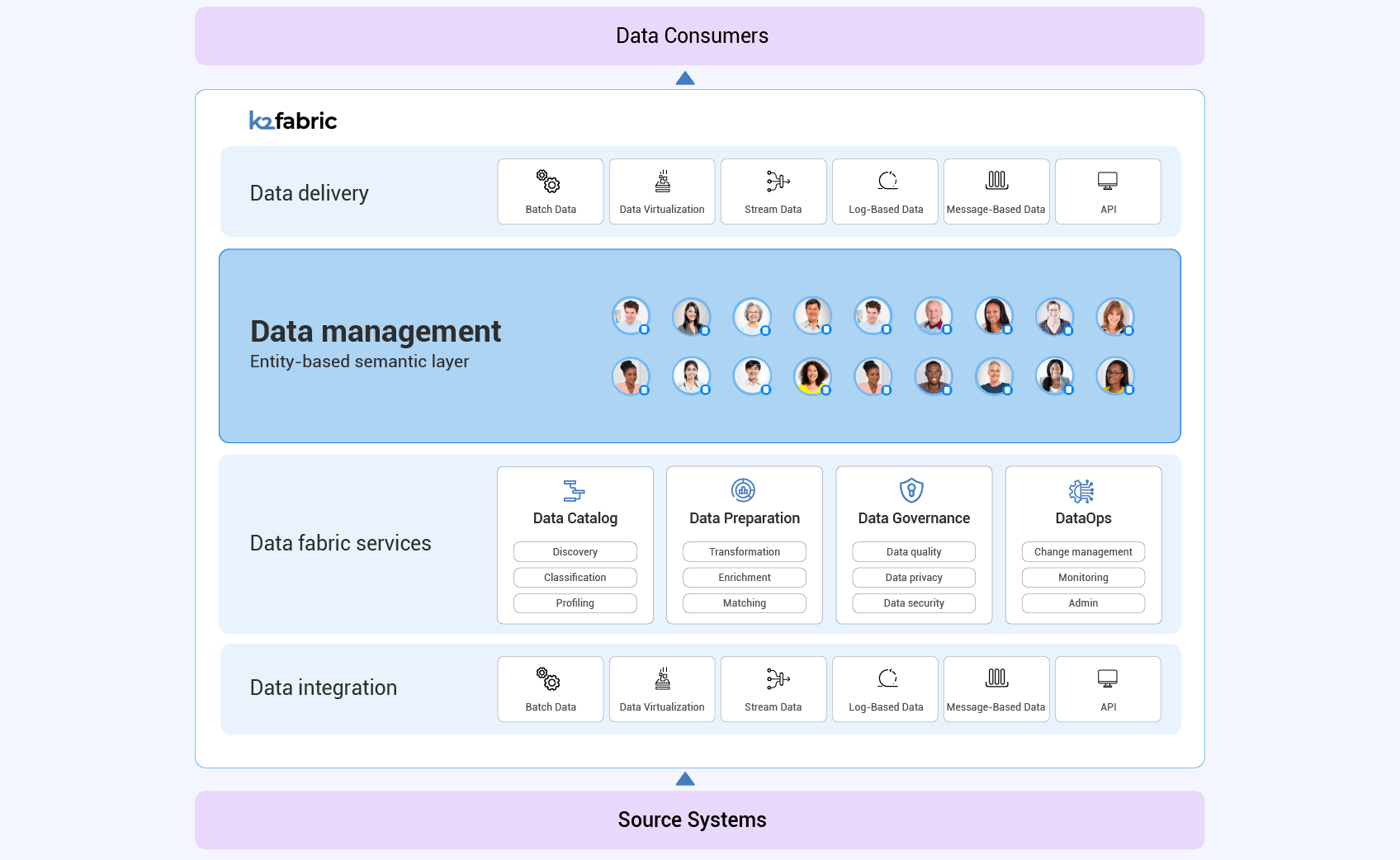
A data fabric that can manage, prepare, and deliver data in real time, creates the ideal data mesh core. Of course, data mesh architecture has its implementation challenges, but these are easily addressed by data fabric:
Data mesh implementation challenges |
How they are handled by data fabric |
---|---|
Requirement for data integration expertise: Data integration across many different enterprise source systems often requires domain-specific expertise in data pipelining. |
Data as a product: When a data product is a business entity managed in a virtual data layer, there’s no need for domains to deal with underlying source systems. |
Federation vs independence: Achieving the right balance between reliance on central data teams and domain independence isn’t simple. |
Enterprise-wide collaboration: Domain-specific teams, in coordination with centralized data teams, build APIs and pipelines for their data consumers, control and govern access rights, and monitor use. |
Batch data and real-time and batch data delivery: Data products must be provisioned to both offline and online data consumers, securely and efficiently, on a single platform. |
Analytical and operational workloads: Data fabric collects and processes data from underlying systems, to supply data products on demand, for offline and online use cases. |
Data Fabric Core Capabilities
Data fabric supports the following key capabilities integrated into a single platform:
-
Data catalog
To classify and inventory data assets, and visually represent information supply chains
-
Data engineering
To build reliable and robust data pipelines for both operational and analytical use cases
-
Data governance
To assure quality, comply with privacy regulations, and make data available – safely and at scale
-
Data preparation and orchestration
To define the data flows from source to target, including the sequence of steps for data cleansing, transformation, masking, enrichment, and validation
-
Data integration and delivery
To retrieve data from any source and deliver it to any target, in any method: ETL (bulk), messaging, CDC, virtualization, and APIs
-
Data persistence layer
To persist data dynamically to enable real-time data processing, search, and analysis.
Data fabric should also address the following key non-functional capabilities:
-
Data scale, volume, and performance
-
Dynamically scale both up and down, seamlessly, no matter how large the data volume.
-
Support both operational and analytical workloads, at enterprise scale.
-
-
Accessibility
-
Support all data access modes, data sources, and data types, and integrate master and transactional data, at rest, or in motion.
-
Ingest and unify data from on-premise and on-cloud systems, in any format – structured or unstructured.
-
The data fabric logical access layer needs to allow for data consumption, regardless of where, or how, the data is stored, or distributed – so no in-depth knowledge of underlying data sources is necessary.
-
-
Distribution
-
Data fabric should be deployable in a multi-cloud, on premise, or hybrid environments.
-
To maintain transactional integrity and data governance capabilities, data fabric needs to support a smart data virtualization strategy.
-
-
Security
-
Where data is persisted, it must be encrypted and masked to meet data privacy regulations.
-
Data fabric should be able to deliver user credentials to the source systems, so that access rights are properly checked and authorized.
-
Data Fabric vs Data Lakes vs Databases
To explain how data fabric enables big data stores to handle operational workloads, a comparison between data fabric, data lakes and databases is useful.
The following chart summarizes the pros/cons of each data store, as it relates to massive-scale, high-volume, operational use cases.
Pros |
Cons |
|
---|---|---|
Data Lake, DWH |
|
|
Relational Database |
|
|
NoSQL Database |
|
|
Data Fabric |
|
Data Fabric Use Cases
In enterprise operations, there are scores of use cases that require a high-scale, high-speed data architecture capable of supporting thousands of simultaneous transactions. Examples include:
Delivering a Customer 360 solution
Delivering a single view of the customer to a self-service IVR, customer service agents (CRM), customer self-service portal (web or mobile), chat service bots, and field-service technicians.
Grounding GenAI apps with real-time data
Injecting unified, fresh data from multi-source enterprise applications into LLMs, using a Retrieval-Augmented Generation (RAG) framework, to generate personalized, trustworthy recommendations.
Complying with data privacy laws
With a flexible workflow and data automation solution, employing data orchestration and data masking tools, for compliance across people, systems, and data – designed to address current and future regulations
Pipelining enterprise data into data lakes and warehouses
Enabling data engineers to prepare and deliver fresh, trusted data – from all sources, to all targets – quickly and at scale.
Provisioning test data on demand
Creating a test data warehouse, and delivering anonymized test data to testers and CI/CD pipelines – automatically, and in minutes – with complete data integrity.
Modernizing legacy systems
Safely migrating data from legacy systems into data fabric, and then using the fabric as the database of record for newly developed applications.
Securing credit card transactions
Protecting sensitive cardholder information by encrypting and tokenizing the original data to avoid data breaches.
Predicting churn, detecting customer fraud...credit scoring, and more
Many operational use cases require data fabric to respond to complex queries in a split second. Therefore, data fabric must include built-in mechanisms for handling:
-
Live data ingestion
Continually updated from operational systems (with millions, to billions, of updates per day)
-
Connectivity to disparate systems
With terabytes of data spread across dozens of massive databases / tables, often in different technologies
-
In-flight data transformation, data cleansing, and data enrichment
To deliver meaningful insights and influence business outcomes in real time
-
A specific instance of a business entity
Such as, retrieving complete data for a specific customer, location, device, etc.
-
High concurrency
To the tune of thousands of requests per second
Data Fabric Advantages
Data fabric offers many advantages over alternative data management approaches, such as master data management, data hubs, and data lakes, including:
-
Enhanced data management
Allowing data to be retrieved, validated, and enriched automatically – without any transformation scripts, or third-party tools -
Expanded data services
Using innovative engines to manage and synchronize data with full support for SQL, and an embedded web services layer -
High consistency, durability, and availability
Meeting enterprise standards, with a trusted database layer and processing engine -
Excellent performance
Relying on an architecture capable of running every query on a small amount of data, and in-memory processing -
Tight security
Eliminating the possibility of mass data breaches, due to a sophisticated, multi-key encryption engine
Data Fabric Benefits
The operational benefits data fabric provides to enterprises include:
- Unified data access
Providing a single, integrated data layer that allows users and applications to access data across different sources and environments seamlessly. This simplifies data access and reduces data silos. - Enhanced data governance
Establishing and enforcing consistent data governance policies across all data sources. This ensures compliance with data privacy regulations (GDPR, CPRA, LGPD,...) and improves data quality and security. - Improved DataOps
Reducing the complexity of data management, automating data discovery and data integration tasks, and optimizing data movement. - Real-time data access and integration
Supporting faster, more informed decision-making, and taking timely action, based on fresh, trusted data. - Reducing GenAI Hallucinations
Grounding LLMs with integrated and fresh enterprise data to deliver more accurate GenAI recommendations that users trust.
Data Fabric Vendors
There are multiple vendors that deliver an integrated set of capabilities to support the data fabric architecture. The top 5 data fabric vendors appear below:
Strengths |
Concerns |
|
---|---|---|
|
|
|
|
|
|
|
|
|
|
|
|
|
|
Data Fabric for Analytics and Operations
It is commonly held that data fabric is built to support big data analytics – specifically trend analysis, predictive analytics, machine learning, and business intelligence – performed by data scientists, in offline mode, to generate business insights.
Data fabric is equally important for operational use cases – such as churn prediction, credit scoring, data privacy compliance, fraud detection, real-time data governance, and Customer 360 – which rely on accurate, complete, and fresh data.
Data teams don’t want to have one data fabric solution for data analytics, and another one for operational intelligence. They want a single data fabric for both.
The ideal data fabric optimizes the field of vision – and the depth of understanding – for every single business entity – customer, product, order, and so on. It provides enterprises with clean, fresh data for offline data analytics, and delivers real-time, actionable data for online operational analytics.
-
The data fabric continually provisions high-quality data, based on a 360 view of business entities – such as a certain segment of customers, a line of company products, or all retail outlets in a specific geography – to a data lake or DWH. Using this data, data scientists create and refine Machine Learning (ML) models, while data analysts use Business Intelligence (BI) to analyze trends, segment customers, and perform Root-Cause Analysis (RCA).
-
The refined ML model is deployed into the data fabric, to be executed in real-time for an individual entity (customer, product, location, etc.) – thus “operationalizing” the machine learning algorithm.
-
Data fabric executes the ML model on demand, in real time, feeding it the individual entity’s complete and current data.
-
The ML output is instantly returned to the requesting application, and persisted in the data fabric, as part of the entity, for future analysis. Data fabric can also invoke real-time recommendation engines to deliver next-best-actions.
Why K2view
K2view is the only data fabric capable of responding to entity-centric data queries in real time, at massive scale, and supporting both operational and analytical workloads.
Here are 5 reasons that K2view has become the data fabric of choice among some of the world’s largest enterprises:
1. A Micro-Database for every business entity
K2view’s patented Micro-Database™ delivers unmatched performance, ease of access, completeness of data, and a common language between Business and IT.
K2view Data Fabric unifies multi-source data for every business entity source into a single Micro-Database, one for every instance of a business entity.
A customer Micro-Database, for example, unifies everything a company knows about a specific customer – including all interactions (emails, phone calls, website portal visits, chats…), transactions (orders, invoices, payments…), and master data – regardless of underlying source systems, technologies, and data formats. In this case, one Micro-Database is managed for every customer.
The Micro-Database may be enriched with new fields that are captured, or calculated on the fly – such as KPIs, consent information, propensity to churn, and more. And it can be easily defined, using auto-discovery, to extract a suggested data schema from the underlying systems.
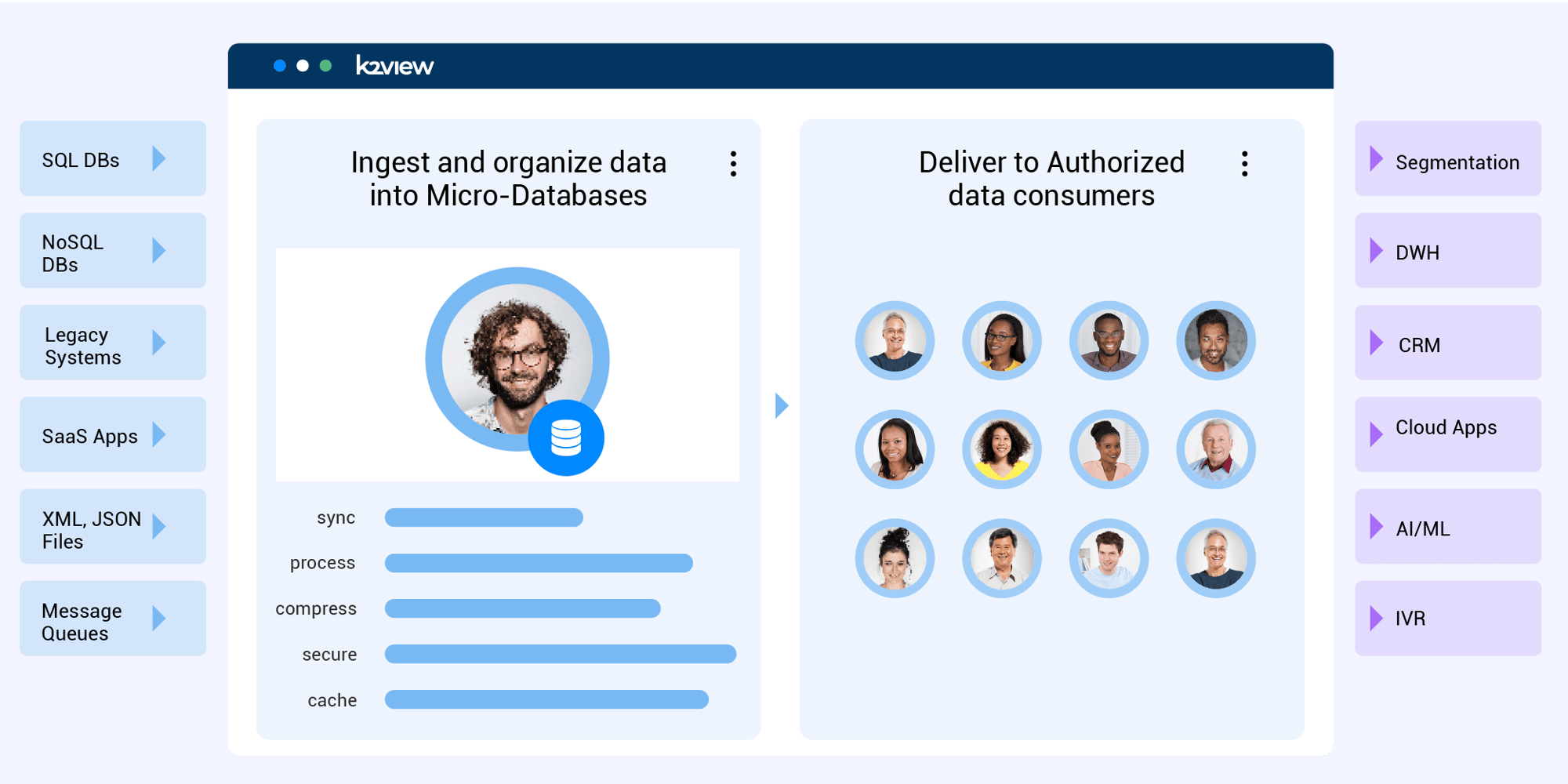
2. Enterprise-scale, unmatched performance, granular security
K2view Data Fabric scales to manage billions of Micro-Databases concurrently by leveraging the following built-in mechanisms:
-
Data sync rules define the frequency and events at which each data element in the Micro-Database is updated from the source systems.
-
Data virtualization rules define which data will be persisted in the Micro-Database, and which will only be cached in memory.
-
Data compression is employed whereby each Micro-Database is compressed by approximately 90%, resulting in lower data transmission costs, and lower hardware requirements.
Furthermore, each Micro-Database is encrypted with its own unique key, so that the data for each entity is uniquely secured. This maintains the highest level of security for data at rest.
K2view Data Fabric can be deployed in a distributed on-premise, on-cloud, or hybrid architecture.
3. Data is integrated and delivered, from any source, to any target, in any style
K2view has developed an operational data fabric that ingests data from any source, in any data delivery style, and then transforms it for delivery, to any target, in milliseconds.
Supported data integration and delivery methods include: ETL, JDBC, Messaging, Streaming, CDC, and APIs.
4. Web services deliver a single view of any business entity to consuming applications
K2view Data Fabric provides a low-code / no-code framework to create and debug web services that expose the data fabric's data to authorized data consumers. Using a visual, drag-and-drop generator, web services can be quickly customized and orchestrated to support any workload. This approach lends itself to treating data as a product and supporting mesh architectures.
Users or tokens that need access to a web service are automatically assigned a role, which defines the level of data access they have. Once a web service is deployed, K2view Data Fabric controls authentication and authorization so that user access is properly restricted.
5. One platform, many use cases
The K2view Data Product Platform delivers a real-time, trusted, and holistic view of any business entity to any consuming applications, data lakes, or data warehouses. The use cases of the platform are therefore numerous, and span many departments in the enterprise.
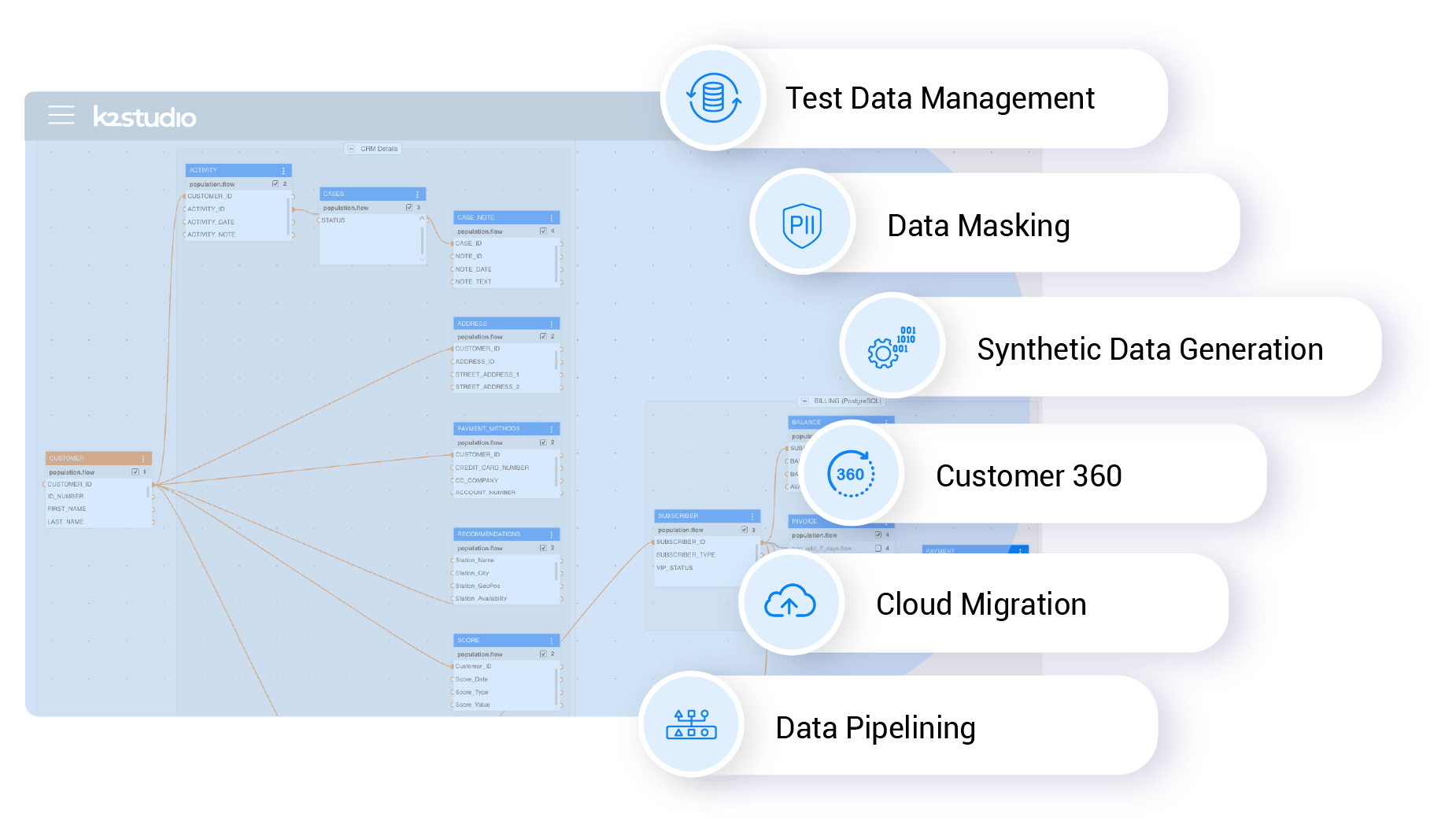
-
A modular, open, and scalable architecture
Data integration, transformation, enrichment, preparation, and delivery – integrated in a single, extensible platform -
Split second, end-to-end, response times
An enterprise-grade platform, built to support real-time operations, with bi-directional data movement between sources and targets -
Data management for operational and analytical workloads
Integrated, trusted data, delivered in real time into consuming applications, or pipelined into data lakes and data warehouses for analytical purposes