Why's it so hard to deliver value
with enterprise GenAI apps?

LLMs lack your
business data
LLMs are generic, trained on dated, publicly available information.

Retraining or fine-tuning
is cost-prohibitive
Retraining LLMs with business data costs millions of dollars every time.

LLMs can generate
false information
LLM hallucinations damage your reputation and customer trust.
Make your data GenAI-ready
K2view extends Retrieval-Augmented Generation (RAG) with a 360° view of your enterprise data. Data products retrieve your structured, operational data in real time, and inject it into LLMs via contextual prompts. K2view powers your GenAI apps to generate accurate recommendations, information, and content – that your users can trust.

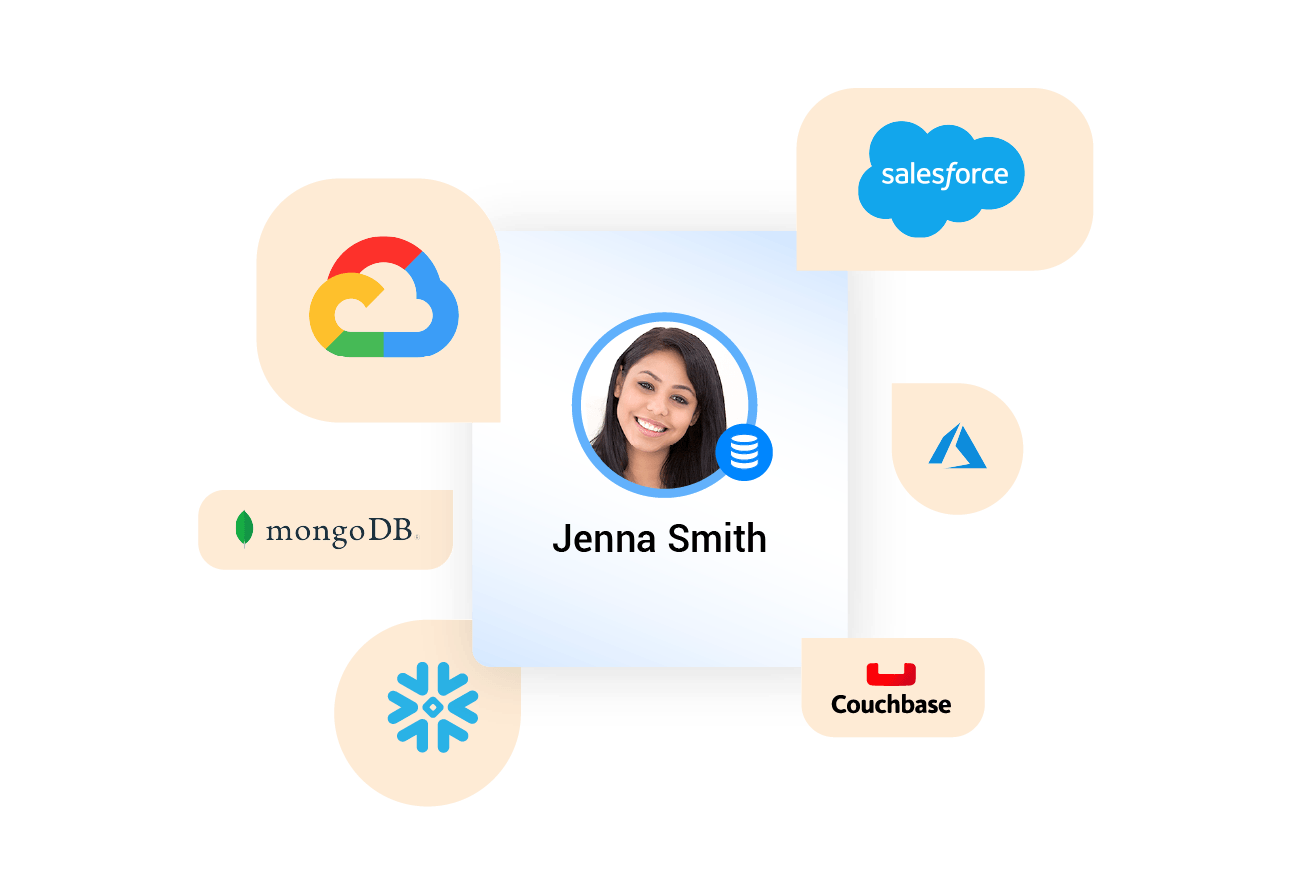
01
Ingest and unify your data
in real time
K2view extends retrieval-augmented generation to include structured enterprise data. Its data product platform ingests and integrates multi-source operational data by business entity (customer, product, supplier, etc.), to create a real-time 360° view of each one. It features:
-
Dozens of built-in connectors, to practically any app or database
-
Code-free matching, deduping, and transformations
-
Inflight data cleansing
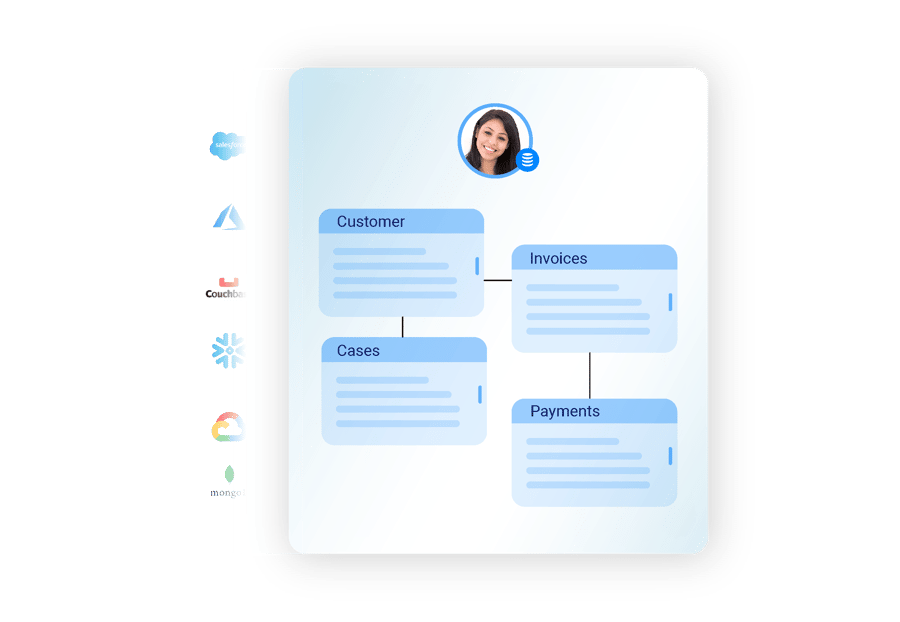
02
Organize your data for GenAI retrieval
K2view Data Product Platform stores the unified 360° data for each entity in its own high-performance Micro-Database™ featuring:
-
Up to 90% compression
-
Continuous sync with underlying source systems
-
Management on commodity hardware, to the tune of billions, concurrently
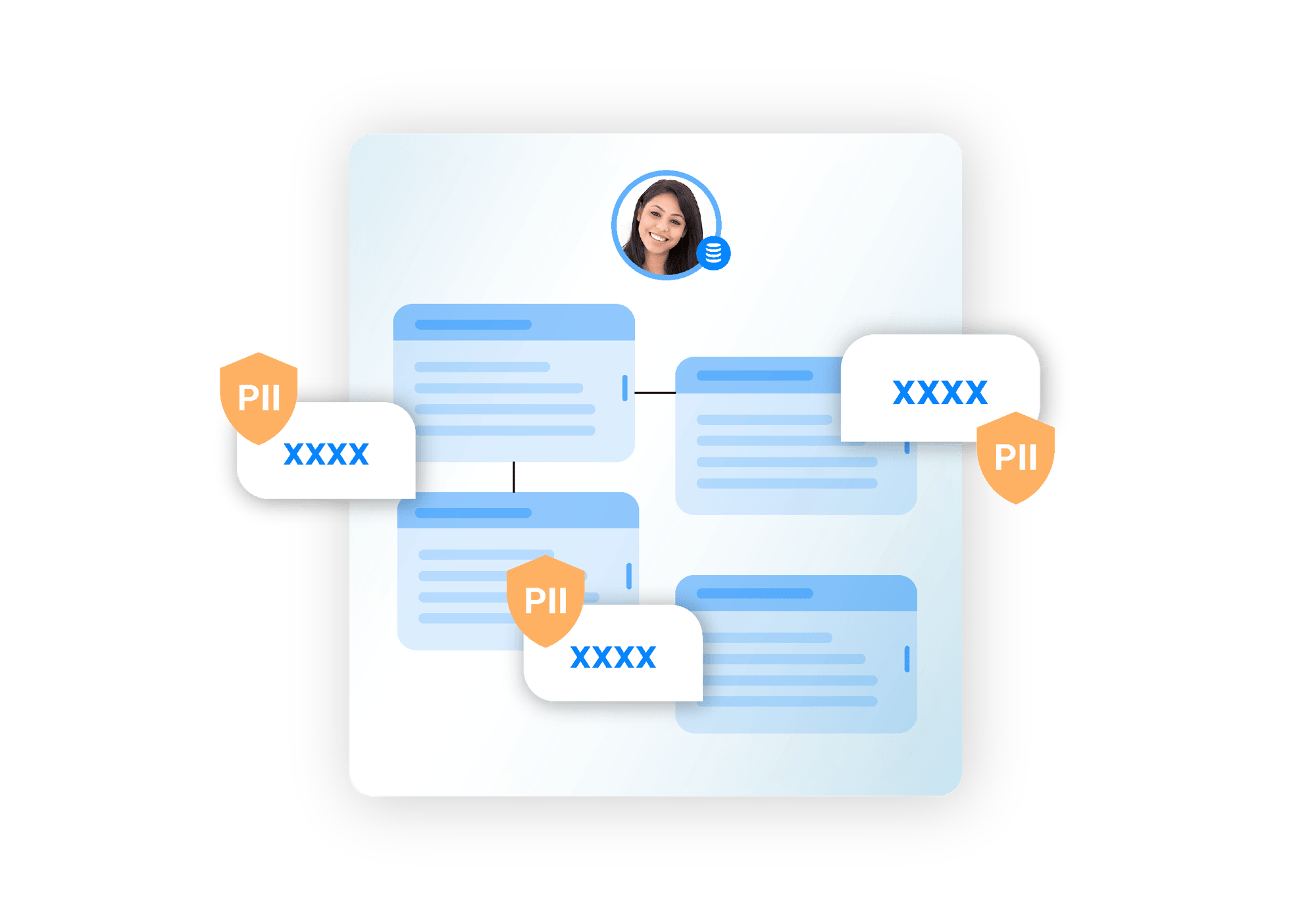
03
Protect your data
K2view ensures that your GenAI app users see only the data they're meant to see.
- Role-based access controls prevent unauthorized access.
- Dynamically data masking protects PII.
- Individually encrypted Micro-Databases nullify mass data breaches.
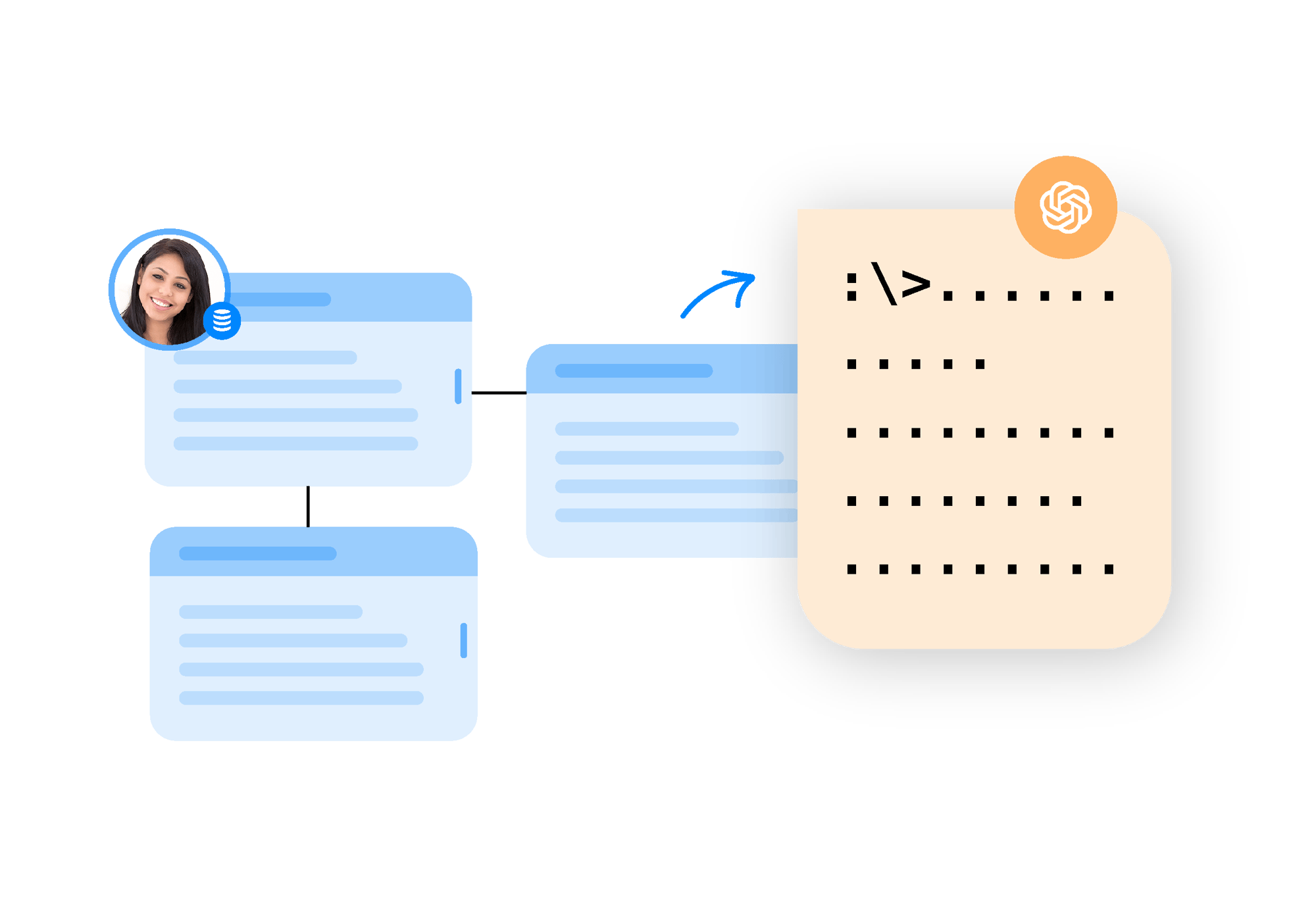
04
Generate context-rich prompts
K2view GenAI Data Fusion creates context-rich prompts that enrich the original user prompt, for more accurate and personalized LLM responses.
-
Micro-Database queries are auto-generated based on user prompts.
-
Queries are answered in milliseconds.
-
User prompts are enriched with relevant context from underlying business systems.
Powering transformative GenAI use cases
with your enterprise data
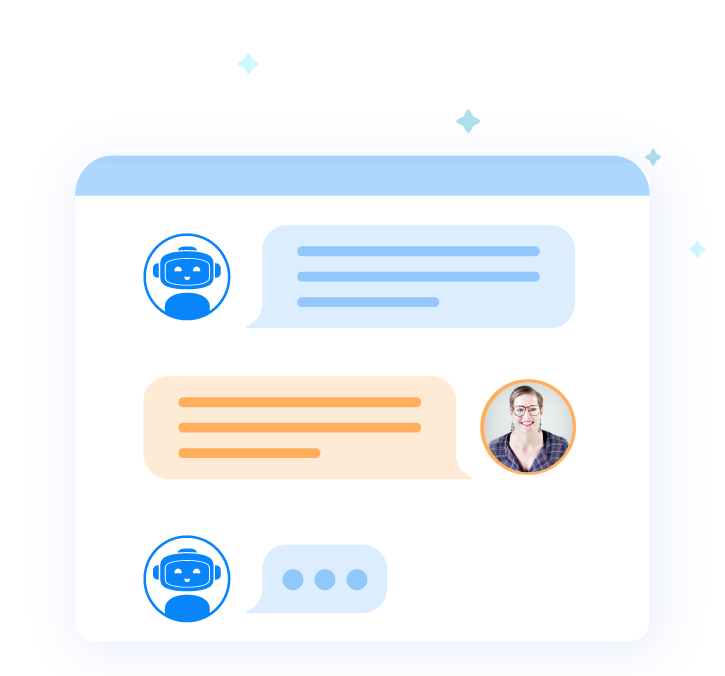
GenAI chatbots
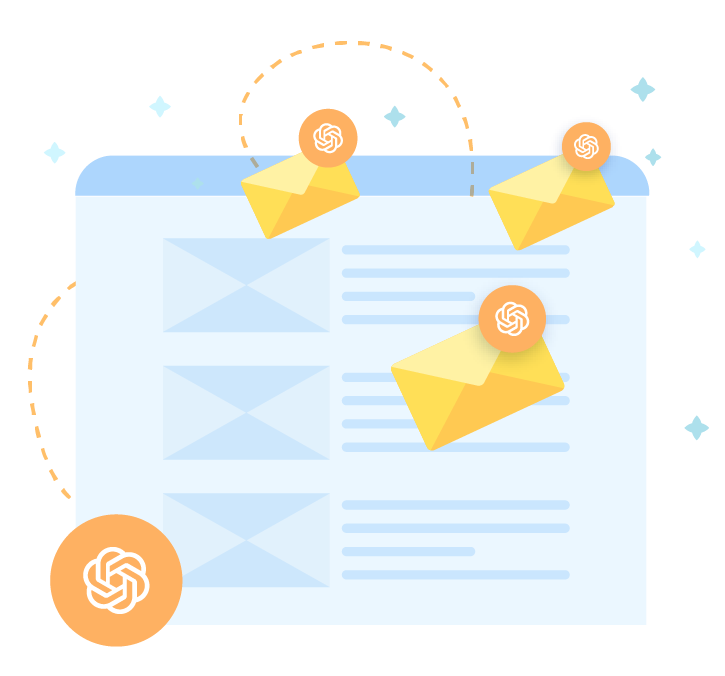
GenAI email marketing
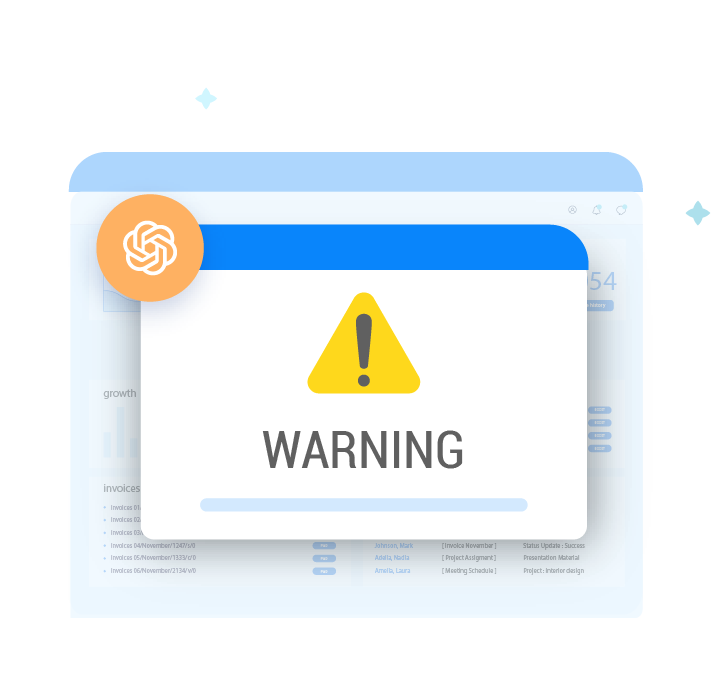
GenAI fraud detection
Frequently Asked Questions
If we have millions of customers, will we have millions of Micro-Databases?
And, how costly will this be to manage?
The answer to the first question is "Yes". The data for every one of your customers is stored in its own high-performance Micro-Database.
K2view is cost-effective because the Micro-Databases are compressed by up to 90% and require minimal CPU for processing. K2view can therefore manage billions of Micro-Databases, concurrently, using commodity hardware.
How are Micro-Databases physically stored?
Micro-Databases can be stored in the private/ public cloud database of your choice, including: Microsoft Azure Blob Storage, Amazon S3, and Google Cloud Storage.
On-prem data stores, such as Cassandra and PostgreSQL, are also supported.
How do Micro-Databases sync with underlying source systems?
The Micro-Database has a built-in, sophisticated data synchronization mechanism that lets you configure user-defined sync rules at the field level.
These sync rules enable you to fully control how and when the Micro-Database is updated from the underlying source systems.
For example, Micro-Database fields whose values rarely change (e.g., address) can by synced infrequently, while quick-changing transactional data may be synced via real-time streaming events (e.g., CDC updates). Yet others can be refreshed on demand, whenever the Micro-Database is queried.
What impact does the Micro-Database sync have on my source systems?
Very little, regardless of the data integration methods used to sync the Micro-Database:
- Streaming, messaging, or CDC methods "push" data updates to the Micro-Database without impacting the source systems at all.
- JDBC queries or APIs, that are invoked against the data sources, "pull" Micro-Database updates for a specific entity. They hardly impact the source systems due to the low compute required to perform a simple query for a single entity.
What is Retrieval-Augmented Generation (RAG)?
Retrieval-augmented generation is a GenAI framework designed to ground LLMs with a company's internal data, typically docs stored in knowledge bases. These docs can include procedures, policies, product documentation, how-to manuals, and more.
Each document's text is converted into numeric vectors that capture textual meanings and inter-relationships . The vectors are stored in a vector database, enabling the LLMs to quickly search for the most appropriate docs to a customer's prompt.
How can RAG leverage structured data for augmenting LLMs?
To leverage the vast amounts of insights and knowledge stored in your structured data sources, the RAG framework must implement a structured data retriever.
The RAG structured data retriever ingests, unifies, cleanses and transforms multi-source enterprise data, and then injects it into the LLM via contextual prompts, in real time. It also employs dynamic data masking and role-based access controls to ensure that users see only the data that they're they're meant to see.
Can RAG be used with both structured and unstructured data?
Certainly.
A RAG workflow can be created to invoke both a structured data retriever and an unstructured data retriever, and merge the responses from both, into a prompt that grounds the LLM.
Data-augmented generation is ideal for customer-centric use cases – like answering questions through chatbots, or generating cross-/up-sell recommendations for call center agents – where customer context is critical for providing accurate and personalized responses from an LLM.
Augments your LLM with your company's internal documents is useful for use cases like competitive analysis, financial analysis, market analysis, generating RFP responses, and more.